Anxiety and self-esteem in cyber-victimization profiles of adolescents
Abstract
This study has two objectives: (1) Identify profiles of victimization in adolescence, depending on the levels of offline or online peer aggression suffered, along with the prevalence of each profile; and (2) Analyse the association of the victimization profiles with adolescents’ social anxiety and self-esteem. The sample was comprised of 3120 adolescents aged 12 to 18 (M=14.03; SD=1.40) from Asturias (Spain), who completed self-report questionnaires about traditional peer victimization and cyber-victimization, as well as social anxiety and self-esteem. We performed descriptive analyses, Latent Profile Analyses, and multivariate analyses of variance. We found a positive, moderate correlation between being a cyber-victim and being a traditional victim, along with four profile types: non-victims (77.8%), mainly cyber-victims (13.5%), mainly traditional victims (4.5%), and dual victims (4.3%). Traditional victims and dual victims exhibited greater social anxiety and less self-esteem than cyber-victims, who exhibited greater social anxiety and less self-esteem than non-victims. Dual victims and traditional victims do not differ in social anxiety and self-esteem. The results contribute to the identification of patterns of victimization in school-age adolescents, and their relationship with social anxiety and self-esteem.
Keywords
Victimization, cyber-victimization, cyberbullying, self-esteem, social anxiety, adolescence
Palabras clave
Victimización, cibervictimización, ciberacoso, autoestima, ansiedad social, adolescencia
Resumen
Este trabajo parte de dos objetivos: 1) Identificar perfiles de victimización en la adolescencia, en función del grado de padecimiento de violencia offline u online por parte de sus iguales, así como la prevalencia de cada perfil; y 2) Analizar la asociación de los perfiles de victimización obtenidos con la ansiedad social y la autoestima del adolescente. Para ello, se aplicaron cuestionarios de autoinforme sobre victimización tradicional entre iguales, cibervictimización, ansiedad social y autoestima a 3.120 adolescentes de Asturias (España), de 12 a 18 años (M=14.03; DT=1.40). Se realizaron análisis descriptivos, análisis de perfil latente y análisis multivariado de la varianza. Se obtuvo una correlación positiva y moderada entre ser cibervíctima y ser víctima de violencia tradicional; y una tipología de cuatro perfiles: no víctimas (77,8%), principalmente cibervictimas (13,5%), principalmente víctimas de violencia tradicional (4,5%) y víctimas duales (4,3%). Cuanto mayor es el nivel de victimización, mayor la ansiedad social y menor la autoestima, siendo más fuerte esta asociación con la victimización tradicional que con la cibervictimización. Tanto las víctimas tradicionales como las duales presentan una mayor ansiedad social y una menor autoestima que las cibervíctimas, y estas que las no víctimas. Víctimas duales y tradicionales no difieren en ansiedad social y autoestima. Los resultados obtenidos contribuyen a identificar patrones de victimización en la adolescencia, y su relación con la ansiedad social y la autoestima.
Keywords
Victimization, cyber-victimization, cyberbullying, self-esteem, social anxiety, adolescence
Palabras clave
Victimización, cibervictimización, ciberacoso, autoestima, ansiedad social, adolescencia
Introduction
Aggression between adolescents via electronic devices, instant messaging programs, and social networks (cyber-aggression) has been the object of growing interest in recent years (González-Moreno et al., 2020). Nowadays there is great social concern about this problem, because of its prevalence and effects (Machimbarrena et al., 2018). However, the nature of the construct, as distinct from traditional aggression, is still open to debate. Authors such as Olweus (2012) and Mehari et al. (2014), considered that these new forms of aggression were essentially no different to traditional forms, and should therefore not be studied in isolation. The underlying problem would be the same, except that it would happen in two different specific contexts (in-person and online). Both forms of victimization are positively correlated and share psychosocial predictors. Rather than being “new” victims, cyber-victims would generally be no different from those suffering from traditional aggression. It is, instead, a new form of aggression which usually overlaps traditional forms (physical, verbal, relational, etc.). In fact, according to authors like Olweus (2012) and Williford et al. (2018), most of the negative impact attributed to cyber-victimization should in reality be attributed to the overlap of traditional forms of aggression.
If an adolescent suffers from traditional aggression, and also from aggression via electronic devices, the latter would add little significant effect. The negative effects of cyber-victimization can only, therefore, be understood in a generally broader context of aggression, including traditional types. This is important, as it points the way towards the most effective prevention. It hints as to whether it is worth prioritizing specific programs for preventing aggression via electronic media (mainly focused on applications, Internet behaviour, and Internet safety) or whether more general programs for preventing aggression between adolescents would have a positive impact on the prevention of cyber-victimization even without specific elements aimed at this type of aggression. Previous studies suggest that programs aimed at reducing traditional aggression, despite not having specific elements about preventing online aggression, also have a positive effect on the prevention of cyber-victimization between adolescents (Gradinger et al., 2016; Salmivalli et al., 2011).
One argument in favour of this hypothesis, is the positive, significant relationship that is usually found between suffering from both types of victimization (online and offline). Some meta-analyses have found a fairly high correspondence between cyber and traditional victimization ( =.40, Modecki et al., 2014; =.43, Gini et al., 2018), although there can be differences depending on age and the context of the sample (Cosma et al., 2020). However, co-occurrence of these two phenomena is not perfect, since being the victim of traditional aggression is generally more prevalent than being a cyber-victim (López-Castedo et al., 2018; Modecki et al., 2014; Sastre, 2016; Viau et al., 2020).
The possible co-occurrence, the overlap between being a victim of offline and online peer aggression in adolescence, has also been examined in studies that have attempted to identify types of victims according to the extent to which they suffer from the different types of aggression (online and offline). The table shown in Álvarez-García (2020) provides a summary of the main studies in this regard. There have been few studies to date, mostly from the USA, with heterogeneous results. The number of different types of victims varies between three and five, with four being the most common. These approaches generally include a type characterized by null or minimal victimization, one or more types characterized by traditional victimization, and one or more types characterized by dual (cyber- and traditional) victimization. The most common typology includes the following four: non-victims, cyber-victims, traditional victims, and dual victims (Beltrán-Catalán et al., 2018; Gini et al., 2019; Mindrila, 2019). “Mainly cyber-victim” is the type with the least consensus. In some studies, cyber-victimization is included in a category of dual or multiple victimization, and in some of these studies distinct levels of dual or multiple victimization are differentiated (Álvarez-García, 2020).
As indicated above, one problematic aspect of studying cyber-victimization in adolescents is identifying the extent to which its relationships with significant variables (risk factors or consequences) are due to the cyber-victimization or whether they can only be understood in the broader context of ordinary victimization, including traditional types. In this study, we focus specifically on social anxiety and self-esteem. Regarding social anxiety, there is a solid body of evidence about the positive relationship between social anxiety and traditional peer victimization in adolescence (Gómez-Ortiz et al., 2017; Pontillo et al., 2019; Romera et al., 2016; Suárez-García et al., 2020). Some studies have concluded that social anxiety increased the likelihood of being a victim (Acquah et al., 2016; Pabian, &Vandebosch, 2016), others that being a victim increased the probability of having social anxiety (Calvete et al., 2018; Silberg et al., 2016; Van-den-Eijnden et al., 2014).
Studies looking at the relationship between adolescent cyber-victimization and social anxiety have also found a positive relationship between the two, noting that social anxiety is a risk factor for cyber-victimization, while there is less evidence that it is a consequence (Pabian & Vandebosch, 2016; Ruíz-Martín et al., 2019; Van-den-Eijnden et al., 2014). In this regard, some studies have found, in line with the hypothesis posed by Olweus (2012), that traditional (and more specifically, relational) victimization has a more negative impact on adolescent social anxiety than cyber-victimization (Landoll et al., 2015; Van-den-Eijnden et al., 2014); and that adolescents who are victims of both types of aggression do not generally present higher levels of social anxiety than those who are victims of only traditional violence (Cañas et al., 2020).
Regarding self-esteem, meta-analyses have shown that there is a reasonably solid body of evidence about its negative relationship with both traditional peer victimization (Tsaousis, 2016) and cyber-victimization (Chen et al., 2017; Kowalski et al., 2014). However, it is not clear which of the two types of victimization has a greater impact on self-esteem, nor the effect of the possible overlap between the two types of victimization on self-esteem. Previous research in Spain has shown that both victimization and cyber-victimization have negative effects on self-esteem, and that the effect is slightly weaker for cyber-victimization (Álvarez-García et al., 2019). Olweus (2012) found traditional victimization to significantly predict self-esteem, while additional cyber-victimization did not significantly contribute. In addition, recent meta-analyses of longitudinal studies have found results that depend on the type of victimization. Traditional victimization and self-esteem demonstrated a bidirectional causal relationship (Van-Geel et al., 2018). In contrast, there was no causal relationship between cyber-victimization and self-esteem (Marciano et al., 2020).
In light of the above, this study has two objectives. Firstly, to identify profiles of victimization in adolescence, depending on the levels of offline or online peer aggression suffered, as well as determining the prevalence of each victim type, in a sample of Spanish adolescents. Secondly, to analyse the association of the victimization profiles with adolescents’ social anxiety and self-esteem. With regard to the first objective, we expect to find the victim typology most often found in previous studies (non-victims, cyber-victims, traditional victims and dual victims) (Beltrán-Catalán et al., 2018; Gini et al., 2019; Mindrila, 2019), with a similar prevalence to these studies for each type of victim.
With regard to the second objective, we expect that the greater the level of victimization, the greater the adolescents’ social anxiety and the lower their self-esteem, and that traditional victimization will be more strongly related to both variables than cyber-victimization.
Material and methods
Participants
The sample was made up of 3120 adolescents (49.4% girls) aged between 12 and 18 years old (M=14.03; SD=1.40) from Asturias (Spain). They attended 19 schools, selected by stratified random sampling from the 145 state-funded secondary schools in Asturias in which Compulsory Secondary Education (CSE) is taught. State-funded schools make up 95.9% of the total number of schools in which CSE is taught in Asturias. To select the sample, we divided the population of schools according to their ownership (public or private-concerted) and selected in each stratum a number of schools proportional to the population.
In Spain, public schools are those in which both their management and their financing are entirely public, and private-concerted schools are those whose management is private, but their financing is partially public. As a result, we selected 11 public schools and 8 private-concerted schools, representing 13.1% of the total. The selected schools were mostly in urban areas with socioeconomically middle-class intake. All of the classes in the four years making up compulsory secondary education were evaluated in each school.
Measures
Traditional victimization at school
In order to determine how often the adolescents suffered from aggression in the physical school environment, we used the Offline School Victimization scale (Álvarez-García et al., 2015). This is a self-report comprised of six items: “Some of my classmates exclude me in games and activities at playtime”, “My classmates avoid me when we have to do group activities in class”, “My classmates make fun of me/laugh at me”, “My classmates say bad things about me behind my back”, “I have been insulted by a classmate to my face”, and “A student at the school has hit me, either in school or outside the school grounds”.
Responses were given on a Likert-type scale with four alternatives (from 1=never, to 4=always). This is a one-dimensional scale ( [6]=19.87; p=.003; GFI=.998; TLI=.992; CFI=.997; RMSEA=.026 [.014, .040]; SRMR=.011), and invariant in relation to gender (configural: [ [12]=27.02; p=.008; GFI=.997; TLI=.991; CFI=.996; RMSEA=.028; SRMR=.012]; metric: [ [18]=62.50; p<.001; GFI=.994; TLI=.982; CFI=.989; RMSEA=.039; SRMR=.036]; scalar: [ [23]=210.69; p<.001; GFI=.996; TLI=.941; CFI=.955; RMSEA=.070; SRMR=.047]), except at its strictest level (strict: [ [32]=606.32; p<.001; GFI=.990; TLI=.871; CFI=.862; RMSEA=.104; SRMR=.129]). The overall score of the scale is the total of the items added together. Total scores range from 6 to 24. High scores reflect high levels of offline victimization at school. The internal consistency of the scale in this study’s sample was moderate (Cronbach’s α=.74; McDonald’s ω=.75).
Cyber-victimization
We used the Cybervictimization Questionnaire for adolescents (CYVIC, Álvarez-García et al., 2017) to determine how often the adolescents had been victims of aggression via mobile phones or the Internet during the three months prior to the survey. It is made up of 19 items and gives scores for four types of cyber-victimization: Impersonation “I have been impersonated in Twitter, Tuenti..., creating a false user profile (photo, personal details...) with which I was insulted or ridiculed”, Visual-Sexual Cyber-victimization “I have been pressured to do things that I didn't want to (whether or not I finally agreed to do them), threatening me with disseminating my intimate conversations or images”, Verbal Cyber-victimization “Someone has made fun of me with offensive or insulting comments on social networks”, and Online Exclusion “They agree to ignore me on the social networks”, along with four additional Visual Cyber-victimization indicators related to being harmed or ridiculed through photographs or videos “They have forced me to do something humiliating, they have recorded it, and then disseminated it to ridicule me”. It uses a Likert-type response format (from 1=never, to 4=always). The overall scale score is calculated by adding the scores for the component items together. Total scores range from 19 to 76. High scores indicate high levels of cyber-victimization. The internal consistency of the overall scale in the sample in our study was moderate (Cronbach’s α=.79; McDonald’s ω=.80).
Social anxiety
To determine the extent to which adolescents reported feeling inhibited or uncomfortable relating to others, especially those who are not close to them, we used a self-report scale with five items (Álvarez-García et al., 2015): “I’m shy and not very talkative, except with my friends”, “I often feel embarrassed saying hello to people”, “I get nervous when I have to be with a group of children that I don’t know well”, “I get uptight if I meet an acquaintance on the street”, and “I find it difficult to get to know new people, to make friends, or to start talking to people that I don’t know”. It uses Likert-type responses with four alternatives (from 1=completely false, to 4=completely true).
This is a one-dimensional scale ( [4]=36.36; p<.001; GFI=.996; TLI=.976; CFI=.990; RMSEA=.049 [.035, .064]; SRMR=.017), and invariant in relation to gender (configural: [ [8]=39.96; p<.001; GFI=.995; TLI=.975; CFI=.990; RMSEA=.049; SRMR=.018]; metric: [ [13]=59.35; p<.001; GFI=.993; TLI=.978; CFI=.985; RMSEA=.046; SRMR=.035]; scalar: [ [17]=114.58; p<.001; GFI=.997; TLI=.964; CFI=.969; RMSEA=.059; SRMR=.037]; strict: [ [23]=159.07; p<.001; GFI=.996; TLI=.963; CFI=.957; RMSEA=.060; SRMR=.054]). The overall scale score is the total of the item scores. Total scores range from 5 to 20. High scores indicate high levels of shyness/social anxiety. The internal consistency of the scale in the sample in our study was moderate (Cronbach’s α=.75; McDonald’s ω=.75).
Self-esteem
To determine the adolescents’ own assessments of themselves, we used a self-report scale with five items (Álvarez-García et al., 2015): “I am happy with my physical appearance”, “I think I am a good person”, “I can do things at least as well as most of my classmates”, “I like how I am”, and “I feel proud of what I do”. The response was collected using a four-point Likert-type scale (from 1=completely false, to 4=completely true). This is a one-dimensional scale ( [4]=35.44; p<.001; GFI=.996; TLI=.979; CFI=.992; RMSEA=.048 [.035, .064]; SRMR=.019), and invariant in relation to gender (configural: [ [8]=32.65; p<.001; GFI=.996; TLI=.983; CFI=.993; RMSEA=.043; SRMR=.017]; metric: [ [13]=61.32; p<.001; GFI=.992; TLI=.980; CFI=.987; RMSEA=.047; SRMR=.040]; scalar: [ [17]=146.79; p<.001; GFI=.999; TLI=.959; CFI=.965; RMSEA=.068; SRMR=.048];strict: [ [23]=243.21; p<.001; GFI=.998; TLI=.948; CFI=.941; RMSEA=.076; SRMR=.076]). The overall scale score is the total of the item scores. Total scores range from 5 to 20. High scores indicate high self-esteem. The internal consistency of the scale in the sample in our study was moderate (Cronbach’s α=.74; McDonald’s ω=.75).
Procedure
Once the measuring instruments and schools were selected, we sought permission from the administration at each school to administer the questionnaires. Each school management team was informed of the study objectives and procedures, its voluntary, anonymous nature, and the confidential treatment of the results. Once schools agreed to participate, we sought informed consent from the students’ parents or guardians, as most of the students were minors. We obtained the consent from the parents or guardians of 76.3% of the students. Before completing the survey, the students were also informed that the study was anonymous and confidential, and that their participation was voluntary. The questionnaires were administered in paper format and it was carried out in the classroom by the research team. Data collection followed the ethical standards of the Ethics Committee of the University of Oviedo (Spain), and the Declaration of Helsinki.
Data analysis
The data were analysed in various phases. The first step was to examine the statistical properties of the observed variables. In addition to the usual descriptive analyses (Pearson correlations, M, SD, kurtosis, skewness), information is provided on the reliability of the measures (Cronbach's alpha and McDonald's omega), as well as on the construct validity and gender invariance. Confirmatory factor analysis was used for the study of construct validity. Information is provided on various types of invariance (configural, metric, scalar, and strict). The criteria for evaluating the quality of fit of the models were: Goodness of Fit Index (GFI), Tucker-Lewis Coefficient (TLI), Comparative Fit Index (CFI), Root Mean Square Error of Approximation (RMSEA), and Standardized Root Mean Square Residual (SRMR). There is evidence of a good fit when chi-square has a p>.05, GFI, AGFI and TLI ≥ .90, CFI≥.95, and SRMR and RMSEA≤.06.
The second step was the latent profile analysis. With the assistance of the Mplus 7.11 statistical package (Muthén & Muthén, 2012), we used traditional victimization and cyber-victimization scores to identify subgroups of victims or victimization profiles. As these two measures were not on the same scale due to their divergent number of items, we standardized them before the analysis (positive values indicate scores above the sample mean and negative values indicate scores below the mean). Given that there are discrepancies in previous studies about the relationship between gender and victimization (Garaigordobil et al., 2020; Gini et al., 2019; Wang et al., 2010), we included gender as a covariate in the estimation of the latent profiles. In order to determine which model best described the relationship between the two victimization measures from the finite set of models, we added successive latent classes to the target model (Nylund et al., 2007). As a rule, the best fitting model will use the optimum number of classes to describe the relationships between the two victimization variables (Galovan et al., 2018).
We used a range of criteria as the basis for selecting the best model. These included the formal Lo-Mendell-Rubin Maximum Likelihood Ratio Test (LMRT), the Parametric Bootstrap Likelihood Ratio Test (PBLRT), the Akaike Information Criterion (AIC), the Schwarz Bayesian Information Criterion (BIC), and the Sample Size Adjusted BIC (SSA-BIC), along with the entropy value and the size of each subgroup or class (Galovan et al., 2018; Nylund et al., 2007). Significant p-values associated with LMRT indicate significant improvement in model fit relative to the solution with one less class. Lower values of AIC, BIC, and SSA-BIC indicate better fit of the model. It is advisable that these criteria complement the information provided by the formal test of conditional fit. Similarly, it is important to note that small classes, although typically considered spurious –often associated with the extraction of too many classes– are occasionally profiles of interest (Hipp & Bauer, 2006).
In order to determine the classification accuracy of the selected model we have taken into account a posteriori probabilities and the entropy statistic. Entropy takes values between zero and one, and the closer it is to one, the more accurate the classification (values higher than .80 indicate good classification quality; Celeux & Soromenho, 1996). Another criterion we considered when selecting the model was the size of the differences, necessarily statistically significant, between the classes with regard to the variables that had been used to create them. We studied these differences via multivariate analysis of the variance, with the classes as the principal factor (obtained using SAVE=CPROBABILITIES in Mplus syntax) and the two victimization variables as dependent variables. Finally, in addition to the statistical indices above, as recommended (Nylund et al., 2007), in selecting the best model we also carefully considered the theoretical interpretability of the classes.
In the same model of Latent Profile Analysis with covariates, we used the AUXILIARY option with the e-setting to test the equivalence of the means of the two outcome variables (i.e., social anxiety and self-esteem) across profiles. If the overall test indicated significant between-class differences, we used posterior probability-based multiple imputations to conduct paired mean comparisons. We used the criteria established by Cohen (1988) to assess the size of the mean differences (small: d=0.20; medium: d=0.50; large: d=0.80). For all analyses, we used maximum likelihood estimation with robust standard error (MLR).
Results
Descriptive statistics
The descriptive statistics and Pearson correlations between the observed variables are shown at Table 1. The greater the victimization (traditional and online), the greater the anxiety and the lower the self-esteem. In the case of self-esteem, the correlation with traditional victimization is slight higher than the correlation with cybervictimization, and both are close to medium in size (r=-.237, d=0.49; r=-.198, d=0.41). However, for social anxiety, while its relationship with traditional victimization is close to medium in size (r=.209, d=0.43), the relationship with cyber-victimization is small (r=.096, d=0.19). There was no appreciable relationship between gender and either form of victimization, although there was a relationship with social anxiety and self-esteem.
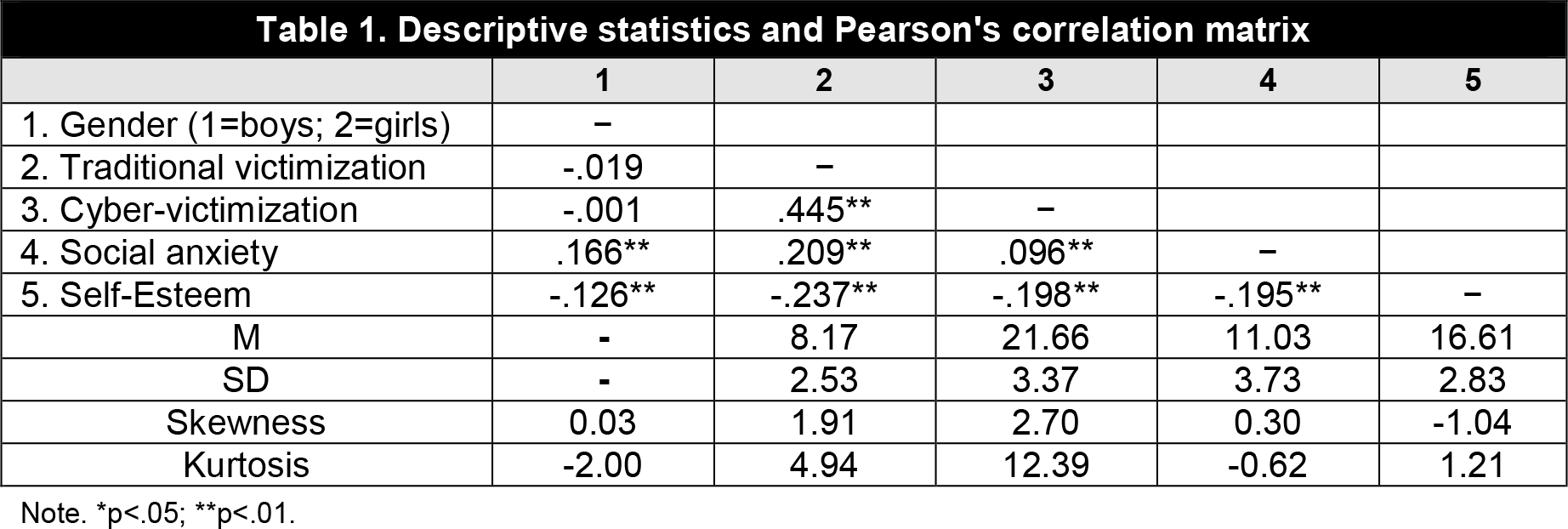
Latent profile analysis
Selection of the best model
Three latent profile models were fitted. The results are shown in Table 2. Although the LMRT-test indicates that the four-class model is statistically no better than the three-class model, the other statistics suggested selecting the four-class model. The AIC, BIC, and SSA-BIC for the four-class model are all lower than the corresponding values for the three-class model. Besides, the entropy is greater in the four-class model, indicating that this model has a better quality than the three-class model. Equally important is the fact that at a theoretical level, there is more basis for a four-class model.
In addition, the two variables were important in differentiating the four profiles: cyber-victimization (F(3,3116)=3888.209; p<.001; d=3.87) and traditional victimization (F(3,3116)=1095.683; p<.001; d=2.05). All of the comparisons between classes in both variables were statistically significant at p <.001. In short, we selected the four-class model over the three-class model because it was statistically better, because it was theoretically valid, and because it demonstrated better quality than the three-class model for classifying the subjects in the classes. The probability of each subject being classified in their assigned class was excellent, both generally (.917) and for each class individually (class 1=.865; class 2=.974; class 3=.889; class 4=.937).
As noted above, the models were fitted using gender as a covariate. The data indicated that gender did not affect the model fit. More specifically, we found that the assignment of subjects to classes did not change with or without gender as a covariate (only one subject was assigned to a different class), and consequently entropy did not change either.
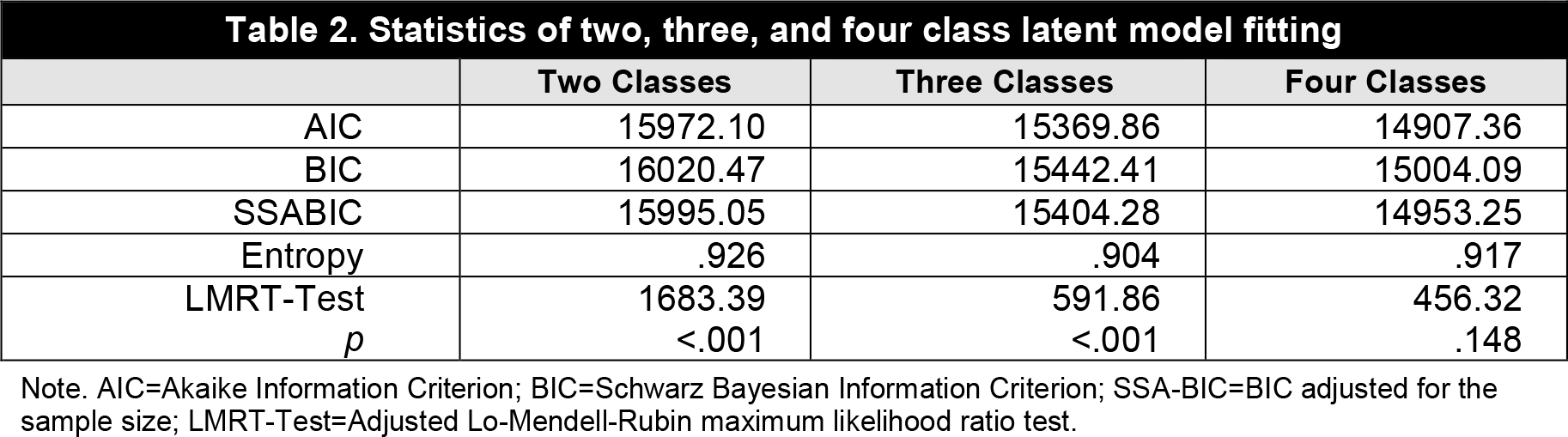
Description of the selected latent class model
The selected latent class model is made up of four profiles of victimization, depending on the levels of offline or online peer aggression suffered (Figure 1).
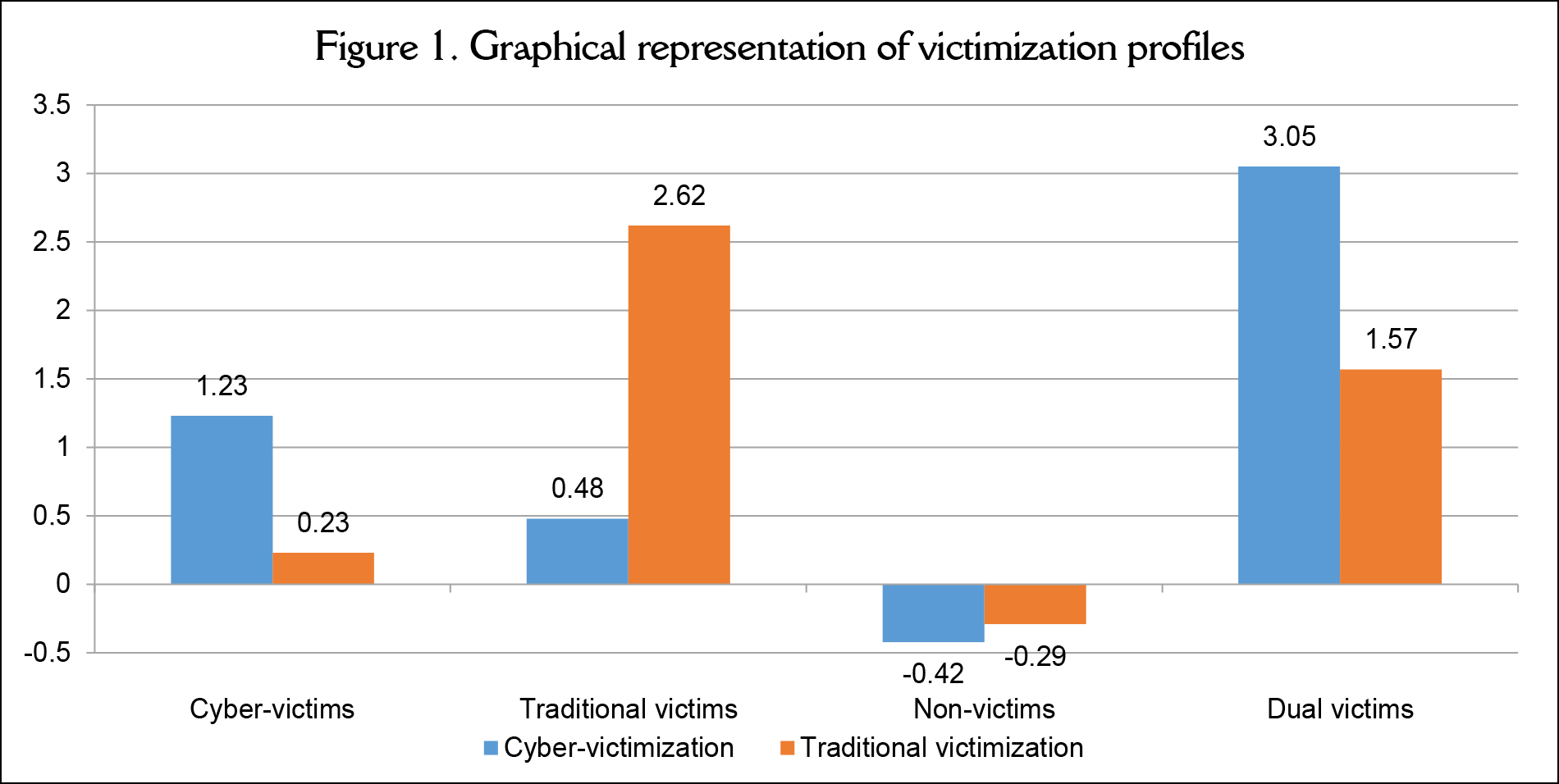
As is shown in Table 3, the largest victimization profile (77.76%) was composed of adolescents who had null or very low scores of any kind of victimization, and it was called “non-victims” (50.3% girls). The next largest group (13.49%) is composed of adolescents who, in general, had low scores of victimization, but that had suffered predominantly from cyber-victimization, so it is called “cyber-victims” (46.1% girls).
The third group (4.46%) had mainly suffered from traditional victimization, so it is called “traditional victims” (39.6% girls). The fourth and smallest group (4.29%) is “dual victims” (53% girls). It is made up of students who had suffered both kinds of victimization significantly over the sample mean.
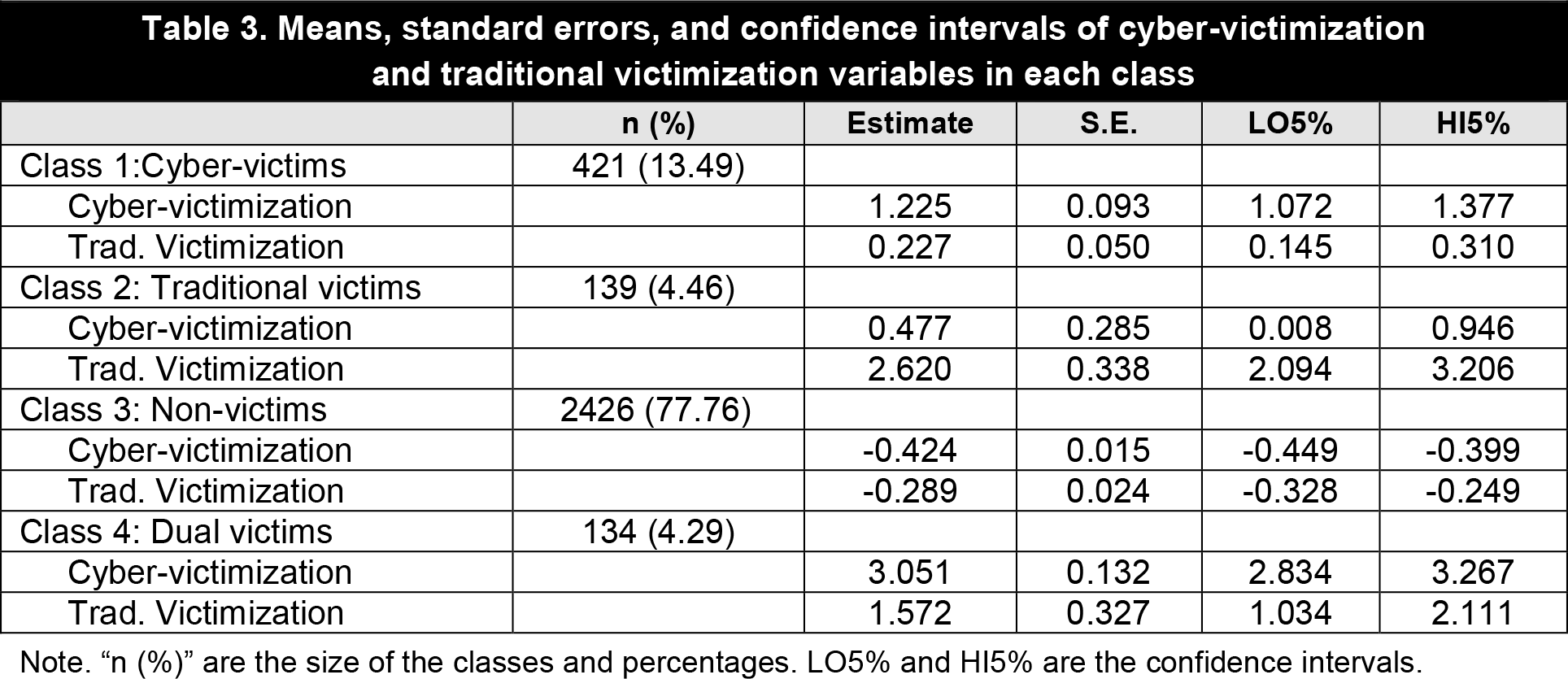
Victimization profiles and health-related outcomes
Non-victims demonstrated the lowest levels of social anxiety and the highest levels of self-esteem. In contrast, traditional victims and dual victims demonstrated the highest levels of social anxiety and the lowest levels of self-esteem (Tables 4 and 5).
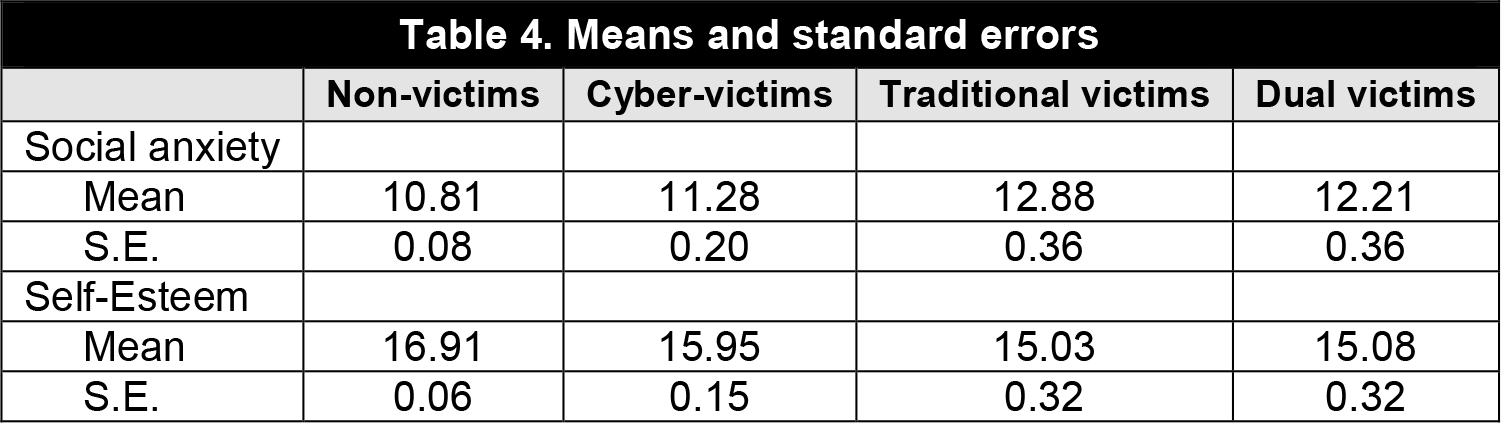
In general terms, the data showed statistically significant differences between the profiles in both social anxiety ( =53.847; p<.001) and self-esteem ( =84.288; p<.001). Considering the multiple comparisons between classes, all the differences were statistically significant, with the exception of the comparison between traditional victims and dual victims in both variables (Table 5).
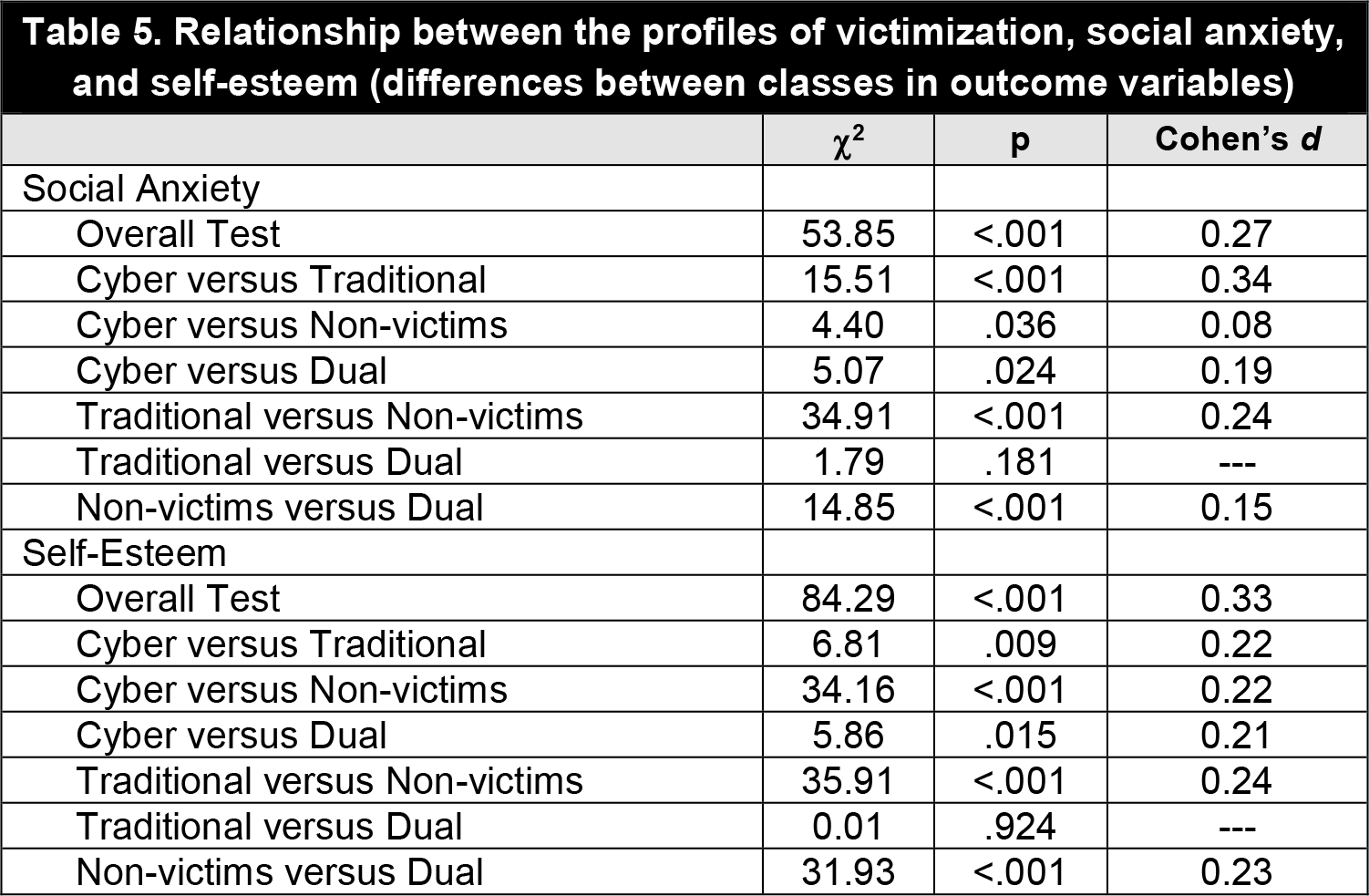
Discussion and conclusions
The first objective of this work was to identify profiles of victimization in adolescence, depending on the levels of offline or online peer aggression suffered, as well as determining the prevalence of each victim type, in a sample of Spanish adolescents. We found, as previous meta-analyses have found (Modecki et al., 2014; Gini et al., 2018), a fairly high positive correlation between being a victim of traditional aggression and being a cyber-victim. The size of the correlation obtained in the present study was very similar to the average correlation obtained in these two meta-analyses. The typology of victimization profiles we found agree with the typology found by Beltrán-Catalán et al. (2018), Gini et al. (2019) and Mindrila (2019), which is what has been found most often in the few published studies on this topic (Álvarez-García, 2020). This typology distinguishes between four types of adolescents: non-victims, cyber-victims, traditional victims, and dual victims. Most of the studies we reviewed (Álvarez-García, 2020) distinguished between non-victims, traditional victims, and dual victims. However, there was less agreement on the category of “cyber-victims”. Our results suggest the importance of considering adolescents who are mainly cyber-victims as a specific type of victims.
As in all of the typologies proposed in the studies we reviewed (Álvarez-García, 2020), in our study the most common profile by far was non-victims. The “dual victims” category had the fewest members, which is the same as in the majority of studies we reviewed which included this category. In our study, the percentage of adolescents suffering predominantly from cyber-victimization was larger than the percentage suffering predominantly from traditional victimization, which contradicts previously published results.
In previous studies where a cyber-victim category has been found, the percentage of students in that category has been significantly smaller (Beltrán-Catalán et al., 2018; Mindrila, 2019) or smaller but not significantly (Gini et al., 2019), than the percentage in traditional victim categories. It is difficult to accurately determine the reason for this disparity, due to the variety of studies in terms of methodology, indicators used, age range and context of the sample (Álvarez-García, 2020). However, a possible reason is that in the present study the adolescents grouped in the “mainly cyber-victimization” class present a less severe general level of victimization than those grouped in the “traditional victimization” class. Maybe that is why the “mainly cyber-victimization” group is more numerous. In the study by Gini et al. (2019), in which the difference in the size of both classes (cyber and traditional victimization) hardly exists, both groups are more similar in overall levels of victimization than in the present work.
Our second objective was to analyse the relationships between the victimization profiles we found, and social anxiety and self-esteem. With social anxiety, our results were consistent with our hypothesis, which was based on prior findings (Gómez-Ortiz et al., 2017; Landoll et al., 2015; Pabian & Vandebosch, 2016; Pontillo et al., 2019; Romera et al., 2016; Ruíz-Martín et al., 2019; Van-den-Eijnden et al., 2014): the greater the level of victimization, the greater the level of social anxiety, with a stronger relationship between social anxiety and traditional victimization than with cyber-victimization.
Our correlation analysis demonstrated that social anxiety was positively, statistically, significantly, associated both with traditional and cyber-victimization. The correlation was stronger with traditional victimization than with cyber-victimization. Analyses of the differences in social anxiety depending on the type of victimization suffered showed that non-victims exhibited the lowest levels of social anxiety. Students who were mainly cyber-victims demonstrated greater social anxiety than non-victims. Students who were mainly victims of traditional aggression demonstrated higher levels of social anxiety than those who were mainly cyber-victims, and therefore than non-victims as well.
Students who suffered from both traditional and cyber-aggression exhibited higher levels of social anxiety than those who were mainly cyber-victims, but there was no statistically significant difference in social anxiety between them and the victims of traditional aggression. This result, in addition to supporting results from previous studies (Gómez-Ortiz et al., 2017; Landoll et al., 2015; Pabian & Vandebosch, 2016; Pontillo et al., 2019; Romera et al., 2016; Ruíz-Martín et al., 2019; Van-den-Eijnden et al., 2014), is consistent with the hypothesis put forward by Olweus (2012), that often cyber-victimization appears linked to traditional victimization, and that in order to understand it, it should be analysed in a broader context of mistreatment, including face-to-face aggression. Our results about self-esteem were consistent with our starting hypothesis, which was based on the prior evidence (Álvarez-García et al., 2019; Chen et al., 2017; Kowalski et al., 2014; Marciano et al., 2020; Tsaousis, 2016; Van-Geel et al., 2018). The greater the level of victimization adolescents suffer, the lower their self-esteem, and this relationship is stronger with traditional than with cyber-victimization.
Our correlation analysis showed that self-esteem was statistically significantly, negatively correlated to both traditional and cyber-victimization. The correlation was slightly stronger with traditional than with cyber-victimization. Analysis of the differences in self-esteem depending on the type of victimization adolescents suffered from showed that non-victims had the highest self-esteem. Adolescents who were mainly cyber-victims exhibited lower self-esteem than non-victims. Mainly traditional victims exhibited lower self-esteem than mainly cyber-victims, and thus also lower than the non-victims. Similar to social anxiety, adolescents suffering from both traditional and cyber-aggression exhibited lower self-esteem than those who were mainly cyber-victims, but they did not differ statistically significantly from traditional victims. This result, in addition to supporting results from previous studies (Álvarez-García et al., 2019; Chen et al., 2017; Kowalski et al., 2014; Marciano et al., 2020; Tsaousis, 2016; Van-Geel et al., 2018), is consistent with Olweus’ (2012) hypothesis that often cyber-victimization appears linked to traditional victimization and to understand it, should be assessed in a broader context of mistreatment, including face-to-face aggression. In fact, Olweus (2012) found that being a traditional victim significantly predicted poor self-esteem, whereas being a cyber-victim did not contribute significantly.
Our study makes a contribution to this field. It is one of the few studies published at an international level to date using mixture modelling in an attempt to identify types of victims in adolescents depending on the extent to which they suffered from online or offline peer aggression. As far as we are aware, it is the first such study in Spain. The results have important theoretical and practical implications. From the theoretical perspective, the study contributes to the consideration of cyber-victimization as a construct in and of itself, which is nonetheless related to traditional victimization. To better understand its risk factors and consequences, it should be examined in the broader context of possible mistreatment, including face-to-face contexts.
From a practical standpoint, our results suggest that, firstly, it is important for early detection of peer aggression to be alert in educational and clinical contexts to students with social anxiety and low self-esteem, because of their higher risk of becoming a victim of peer aggression. Secondly, in terms of prevention and treatment, it is important to work on self-esteem and social interaction skills. Lastly, our results do not support the idea that it is sufficient to have general preventive programs against mistreatment among adolescents in order to prevent cyber-victimization. There is an overlap, and the attitudes, values, and skills that are worked on to prevent aggression in face-to-face contexts can have a positive effect on preventing aggression in virtual contexts (Gradinger et al., 2016; Salmivalli et al., 2011). Nevertheless, there are some adolescents who are mainly cyber-victims, and aggression via virtual contexts has some particular characteristics that mean it is advisable to work on it specifically (Del-Rey et al., 2018; Hinduja & Patchin, 2015).
Although this study does represent a contribution to the field, it is important to recognize some limitations. Firstly, the data were collected via self-reports, which can often produce response biases such as social desirability -although we attempted to minimize this during the process by ensuring the anonymity and confidentiality of results. Secondly, we used a large sample which was representative of the population it was taken from, but limited in age and geographical area. Any generalization of these results to other ages or other geographical areas should be done with caution. It would be advisable to replicate this study in other samples to examine the external validity of the results. (1)