When negativity is the fuel. Bots and political polarization in the COVID-19 debate
Abstract
The contexts of social and political polarization are generating new forms of communication that affect the digital public sphere. In these environments, different social and political actors contribute to extreme their positions, using bots to create spaces for social distancing where hate speech and incivility have a place, a phenomenon that worries scientists and experts. The main objective of this research is to analyze the role that these automated agents played in the debate on social networks about the Spanish Government’s management of the global COVID-19 pandemic. For this, “Social Big Data Analysis” techniques were applied: machine learning algorithms to know the positioning of users; bot detection algorithms; “topic modeling” techniques to learn about the topics of the debate on the web, and sentiment analysis. We used a database comprised of Twitter messages published during the confinement, as a result of the Spanish state of alarm. The main conclusion is that the bots could have served to design a political propaganda campaign initiated by traditional actors with the aim of increasing tension in an environment of social emergency. It is argued that, although these agents are not the only actors that increase polarization, they do contribute to deepening the debate on certain key issues, increasing negativity.
Keywords
COVID-19, political bots, political polarization, digital propaganda, public opinion, social networks analysis
Palabras clave
COVID-19, bots políticos, polarización política, propaganda digital, opinión pública, análisis de redes sociales
Resumen
Los contextos de polarización social y política están generando nuevas formas de comunicar que inciden en la esfera pública digital. En estos entornos, distintos actores sociales y políticos estarían contribuyendo a extremar sus posicionamientos, utilizando «bots» para crear espacios de distanciamiento social en los que tienen cabida el discurso del odio y la «incivility», un fenómeno que preocupa a científicos y expertos. El objetivo principal de esta investigación es analizar el rol que desempeñaron estos agentes automatizados en el debate en redes sociales sobre la gestión del Gobierno de España durante la pandemia global de COVID-19. Para ello, se han aplicado técnicas de «Social Big Data Analysis»: algoritmos de «machine learning» para conocer el posicionamiento de los usuarios; algoritmos de detección de «bots»; técnicas de «topic modeling» para conocer los temas del debate en la red, y análisis de sentimiento. Se ha utilizado una base de datos compuesta por mensajes de Twitter publicados durante el confinamiento iniciado a raíz del estado de alarma español. La principal conclusión es que los «bots» podrían haber servido para diseñar una campaña de propaganda política iniciada por actores tradicionales con el objetivo de aumentar la crispación en un ambiente de emergencia social. Se sostiene que, aunque dichos agentes no son los únicos actores que aumentan la polarización, sí coadyuvan a extremar el debate sobre determinados temas clave, incrementando la negatividad.
Keywords
COVID-19, political bots, political polarization, digital propaganda, public opinion, social networks analysis
Palabras clave
COVID-19, bots políticos, polarización política, propaganda digital, opinión pública, análisis de redes sociales
Background and introduction
The polarisation that occurs in social and political debates on social networks such as Twitter or Facebook has become an increasingly relevant phenomenon for the social sciences. This is not only because it can create a divide between the parties involved in public debate, but also because this divide occurs as a consequence of strategies such as “incivility” or “flaming”, which are based on hatred, the discrediting of one of the parties, name-calling, etc. In short, polarisation is not only relevant because of its consequences, but also because of the emergence of "modes of communication" that can generate a state of "failed communication."
Generally speaking, political polarisation is associated with selective exposure to information. It is believed that this limitation facilitates the development of extreme values, attitudes, and political stances, or is at least based on the strengthening of the earlier stances of the persons involved in the debate (Prior, 2013). In this sense, digital social networks favour polarisation by allowing greater control over the type and the sources of information (Sunstein, 2001, 2018). In this context, polarisation experts have warned about how important different social and political players and stakeholders are in the development of polarisation processes. It has been shown that when extreme positions are adopted by political leaders, it causes a ripple effect that ends up influencing the position of their followers. When leaders slant or present biased information or interpretation of a media event, such bias makes it harder for their followers to understand representatives of opposing views (Boxell et al., 2017).
Our work aims to explore this area by focusing on the role of an agent that can be key in polarisation processes: the "bots". Although the literature on the interference of this type of non-human agent in social, political, and electoral processes is extensive (Ferrara et al., 2016; Howard et al., 2018; Keller & Klinger, 2019), the study of their role in polarisation processes is less so. The question arises as to whether “bots” are contributing to the polarisation of political debate during times of social upheaval. In this sense, our main objective is to study to what extent and by means of what strategies these agents create or exaggerate the processes of political polarisation in debates that take place on digital social networks. To achieve this objective, we are using as a case study the public debate that took place on social networks regarding the Spanish government's management of the COVID-19 global pandemic health crisis during the first few months.
Digital political polarisation
Of course, there is no universally accepted definition of polarisation. Abramowitz (2010) sees it as a process that gives greater consistency and strength to the attitudes and opinions of citizens and parties; and others such as Fiorina and Abrams (2008) state that it is the distancing of people's views in a given political context. Similarly, experts are divided between those who argue for the centrality of an ideological polarisation and others who support the emergence of an emotional polarisation (Lelkes, 2016). However, there are polarisation processes that might be "to be expected" (Sartori, 2005); for example, in two-party contexts, especially when the electoral system is presidential. That is, when two candidates face each other in an electoral process, it is to be expected that their followers will be structured around two poles (generated by each of the candidates). The same can happen when a debate arises around an issue that has two clearly defined positions (e.g. for or against). Polarisation studies have recently focused their attention not so much on the study of the formation of poles, but on those aspects that lead towards the process of negative public debate. Numerous studies suggest that not being in contact with multiple and/or reliable information, intentionally negativised and/or incomplete information is what transforms polarisation into a negative process. Authors such as Prior (2013) state that combining selective exposure to information with negative polarisation results in the entrenching, or radicalisation of, the starting positions of the followers on both sides.
Experts have highlighted different mechanisms to explain this process. From a methodological and experimental individualistic point of view, Taber and Lodge (2006) initiated a field of analysis on the psychological and rational components of the selection of those pieces of information that best matched the individuals' prior conceptions. In this sense, humans tended to filter information in such a way as to identify that which reinforced their previous conceptions or emotional dispositions as more relevant or truthful. Sunstein (2001; 2018) highlights the significant role played by the internet and digital social networks in the polarisation process. From this point of view, the internet offers the possibility of selecting the sources of information to which people are exposed more precisely, as well as the people with whom they choose to debate. Finally, different authors show how major political players are the key agents in polarisation by creating a ripple effect of their potentially biased and/or negative messages (Allcott & Gentzhow, 2017). We know that people's levels of polarisation are closely linked to the membership of certain social groups and the consumption of certain political information (Boxell et al., 2017). This circumstance may be a consequence of the polarisation they receive from the main political agents: parties, organisations, media, etc., which could lead to the contagion effect. The danger of this contagion effect is what Lelkes (2016) calls "emotional polarisation", a process in which citizens tend to radicalise their emotions and/or affections on various issues. They also become fixed on them, following the polarised discourses of political parties and public representatives. In a similar vein, Calvo and Aruguete (2020: 60-70) believe that emotional polarisation on the networks constitutes "a fiery defence of one's own beliefs in the face of the other's communicational objectives" and affirm that "hating the networks is an emotional, cognitive and political act".
Recent studies (Mueller & Saeltzer, 2020) also refer to this network contagion being caused by messages that evoke negative emotions and they suggest that negative emotional communication freely arises as a result of strategic campaigns (Martini et al., 2021). In this context, "incivility" emerges as a communicative strategy which, resulting from the generation of negative emotions through insult or social discrediting, attempts to exclude the adversary from public debate (Papacharissi, 2004). Our work is based on this latter theoretical context, in which polarisation is understood to be the process of extension of the attitudes of political leaders or, as in this case, of the digital tools that play central roles in the debate.
The role of "bots" in digital propaganda
Advanced AI and micro-targeting systems mobilise users through social networks. Specifically, in digital political communication, these propaganda tactics go beyond for-profit fake news and conspiracy theories because they involve the deliberate use of misinformation to influence attitudes on an issue or towards a candidate (Persily, 2017). Among these propaganda strategies are political "bots", which are social media accounts controlled in whole or in part by computer algorithms. They create automatic content to interact with users, often by impersonating or mimicking humans (Ferrara et al., 2016). The main purpose is to break the flow of debate in the networks by denigrating opponents or users who have opposing views (Yan et al., 2020). Some authors point out that the use of "bots" is not always linked to malicious purposes, highlighting as an example the usefulness of Twitter in informing the population about the risks of the COVID-19 pandemic, in disseminating accurate breaking news, and in urging citizens to stay at home (Al-Rawi & Shukla, 2020).
However, while the use of “bots” in pandemics is still an under-researched field of study, there is already empirical evidence that they have been used to promote conspiracy theories in the multimedia political sphere regarding the dissemination of controversial and polarised messages (Moffit et al., 2021). In contrast to social “bots” of an inclusive nature, research highlights political “bots” whose function is to disseminate messages containing negative emotions (Stella el al., 2018; Neyazi, 2019; Yan et al., 2020; Adlung et al, 2021), to spread fake news on a large scale, (Shao et al., 2018; Shu et al., 2020; Yan el al., 2020) and to draw on users' private information for partisan political purposes (Boshmaf et al., 2013; Persily, 2017; Yan et al., 2020).
Several authors warn that, in an increasingly polarised and troubled network environment, political “bots” increase the level of vulnerability of users because they are better able to segment them and target their propaganda (Stella et al., 2018; Yan et al., 2020). Price et al. (2019) also highlight the difficulties that the various “bot” detection tools face due to the constant developments in their ability to improve and modify behaviour. The rise of political tension in the public digital sphere is also linked to disinformation campaigns on social media such as "astroturfing": an activity initiated by political players on the internet. This is strategically manufactured in a top-down manner, mimicking bottom-up activity by autonomous individuals (Kovic et al. 2018:71). They consist of a set of robots coordinated by grassroots activists who emulate ordinary citizens and act independently. They have the potential to influence electoral outcomes and political behaviour by positioning themselves for or against various causes (Howard, 2006; Walker, 2014; Keller et al., 2019). Also known as "Twitter bombs" (Pastor-Galindo et al., 2020) or "cybertroops" (Bradshaw & Howard, 2019), they operate by disseminating comments that are very similar to each other and consistent with the objective of the propaganda campaign (Keller et al., 2019). Often, the features that characterise this type of robotic messaging are the use of false information, uncivil language and hate messages against minority or opposing opinion groups. Moreover, attempts are made to harrass and exclude these groups from the debate (Keller et al., 2019; Santana & Huerta-Cánepa, 2019).
Beyond the damage that this practice wreaks on the natural flow of conversations on social networks, the main problem is that it often leads to the process of strong polarisation. When these dynamics involve extreme positions that prevent dialogue, it is called centrifugal polarisation (Sartori, 2005) and can pose a threat to democracy (Morgan, 2018). Papacharissi (2004) refers to this type of polarisation as "incivility" and specifies that it involves the use of inappropriate, insulting, or demeaning language. Messages that invade the polarised online debate and infringe on personal freedoms or the freedoms of certain social groups (Rowe, 2015). Sobieraj and Berry (2011) refer to “outrage” as a type of political discourse that aims to provoke visceral audience responses such as fear or moral outrage through the use of exaggerations, sensationalism, lies, inaccurate information or partial truths that affect specific individuals, organisations or groups.
The literature points to the fact that hate speech in cyberspace can be fuelled by non-human agents such as social bots, leading to a global problem that was exacerbated by the current COVID-19 crisis (Uyheng & Carley, 2020). We understand that this use of harmful AI tools polarised and increased "incivility" in the political debate regarding the pandemic. The polarisation derived from this health crisis has been the subject of study on platforms such as Youtube (Serrano-Contreras et al. 2020; Luengo et al., 2021). We believe that the presence of "bots" in the debates that took place during the health crisis is an area that needs to be investigated further. Our hypothesis is that these agents are not the only key players in the polarisation process but that they do use polarised situations to heighten the debate by including a greater degree of negativity, particularly on certain key issues. We therefore believe it necessary to investigate the involvement of these agents and the effect they had on Twitter during the “State of Alarm.”
Material and methods
Our database is comprised by tweets downloaded throughout the entire “State of Alarm” period of lockdown that was imposed in Spain. To achieve our objective, we applied "Social Big Data Analysis" techniques such as machine learning algorithms to find out the stance of users on the network towards the Spanish government, algorithms for detecting "bots,” “topic modelling” techniques to ascertain the topics of debate on social networks, and sentiment analysis.
Data source and cleansing
The data was downloaded from the Twitter API via R-Studio with the "rtweet" library (Kearney, 2019). The data was downloaded according to a set of keywords composed of the names of the accounts of the main political parties in Spain and those of their political leaders, and they also included the words "state of alarm", "coronavirus" and "COVID". The database that was generated covers the period of 16 March 2020 to 29 June 2020. Data were downloaded in 5 different batches, during the first week of each of the phases of the “State of Alarm”, to cover the whole period. By the end, 4,895,747 messages were collected.
A cleansing of the data was carried out to remove any downloaded messages that were not relevant to the aim of the study. For this purpose, the "machine learning" methodology was applied. Also, because of how lively the debate was on the networks, many algorithms trained as batches were downloaded. For this purpose, a simple random sample of 1,500 tweets per batch was generated for manual pre-coding by a trained expert. This coding consisted of labelling the messages as "belongs" or "does not belong" to the target of the study. During this process, machine learning algorithms were applied, with lineal Support Vector Machines (SVMs) showing the best performance, finding an average inter-batch accuracy of 0.8 and an average F-measure of 0.768. To carry out this task, text processing was applied to ensure the correct compatibility with machine learning algorithms. Firstly, the content was “tokenised”, separating any given tweet into all the words it included. Secondly, words whose content did not provide relevant “stopwords”, such as determiners, prepositions, etc., were eliminated. Finally, a tf-idf ("term frequency - inverse document frequency") matrix was constructed as input for the machine learning algorithms, where each row represented a tweet, and the columns represented all the words that appeared in the body. Following the application of SVM-linear, a total of 1,208,631 messages corresponding to the study target, posted by 469,616 users, were highlighted.
Detection of “bots”
To detect and classify users as "bots" or "non-bots", the algorithm proposed by Kearney (2019) called "tweetbotornot" in its "FAST-gradient boosted" version, incorporated in the R-Cran statistical package "tweetbotornot", was applied. Also, to be as conservative as possible, only users in the highest quartile of probability of being "bots" were actually identified as "bots.” We believe it is vitally important to maintain this conservative stance because it is preferable to detect fewer "bots" than to include any real users in the "bot" category.
Polarisation measurement
To measure polarisation on social networks, the measurement means of Guevara et al was applied (2020), it is based on fuzzy logic, known as JDJ. The authors go by the premise that reality is not clearly defined one way or the other, but rather that there are different nuances in people's attitudes. It is understood that while a given individual may be, for example, a supporter of one political party, this does not necessarily mean that they may not still agree with some of the proposals of other, different political parties. In this way, instead of looking in a clear-cut way at a person's attitudinal score, the degree to which that person's attitude belongs (or is close) to the poles of the attitudinal axis being measured is computed. In this way, the position of an individual towards the extremes of a variable is considered simultaneously. Thus, the risk of polarisation between an individual "i" and an individual "j" is understood as the joint consideration of the following scenarios:
-
How close the individual "i" is to pole A and how close the individual "j" is to pole B.
-
How close the individual "i" is to pole B, and how close the individual "j" is to pole A.
Thus, the total polarisation of a population set is the sum of all the possible comparisons between the individuals that compose it.
-
Given a variable X.
-
Each individual i∈N.
-
XA,XB are the poles of X and μXAμXB the membership functions of an individual to the poles: μXAμXB:N→[0,1] are functions, and for each i∈N μXA (i) and μXB (i)y are the membership functions of the individual "i" to both poles.

Where, is an “overlapping” aggregation operator and is the grouping function. In this study, the product has been used as the “overlapping” operator and as the maximum grouping function. This measure presents its maximum value when 50% of the population has a maximum degree of membership to pole A and a null degree of membership to pole B, and the other 50% of the population has a maximum degree of membership to pole B and a null degree of membership to pole A. On the other hand, a null level of polarisation is found not only when 100% of the population has the same attitude level, but also when this value is situated around an extreme, this scenario being the one that presents the greatest distance from the maximum value of polarisation. Since the above equation gives as a result the sum of the polarisation risk for all possible combinations of pairs of individuals, the following calculation is made to facilitate its interpretation:
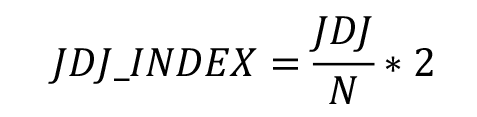
Where is the total number of individuals. In this way, the measurement shows its minimum value at 0 and its maximum value at 1. Guevara et al. (2020) provides a detailed comparison of this proposal with other measurements in the literature.
Stopping topics
To detect the discourse topics, present in the downloaded messages, the Latent Dirichlet Allocation (LDA) algorithm, present in the R package called "topicmodels" (Grün & Hornik, 2011), was used. This algorithm is based on creating distances between words according to their occurrence together. The algorithm has the particularity of indicating the number of topics a priori, so that for the correct determination of the number of topics, measures of semantic coherence by topics can be applied. It is also recommended that an expert scan the content to determine the appropriate number of topics.
Sentiment Analysis
Sentiment analysis dictionaries were used to detect the amount of negative or positive content present in the digital debate. The Afinn dictionary was used (Hansen et al., 2011), consisting of 2,477 words, scored from most negative to most positive, on a scale of five to five.
Analysis and results
Classification of messages as "for" or "against" the government
Firstly, machine learning algorithms were applied to encode a given message as "for" or "against" the government. Here, too, the support vector machines showed better results (Table 1). The results indicate satisfactory performance levels, allowing for the correct automatic classification of all the messages present in the database.
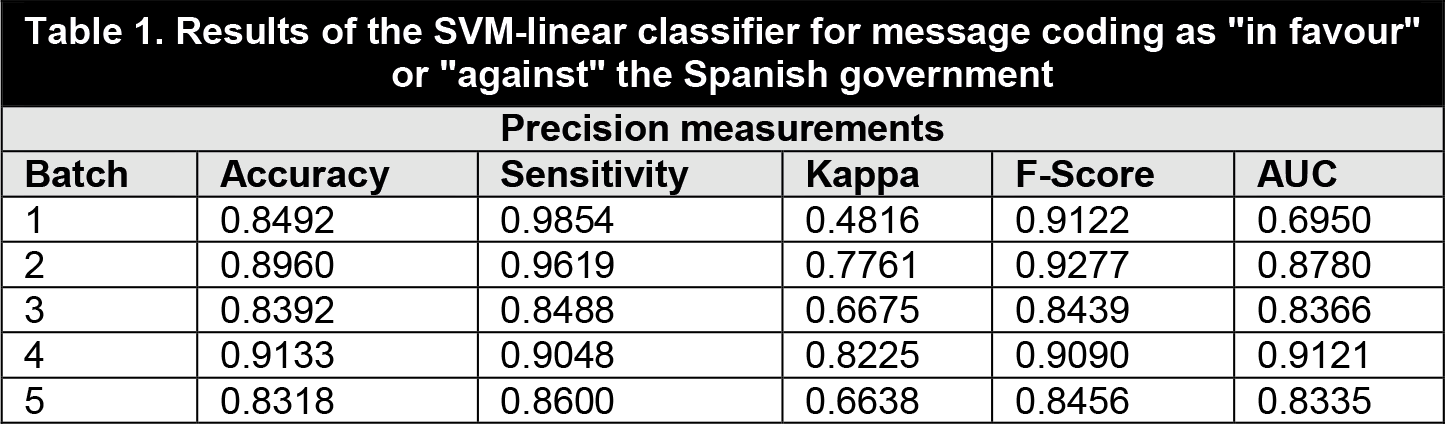
Detection of “bots”
The bot detection algorithm was then applied to identify automated accounts as "bots". The criterion used corresponded to classifying as a "bot" those users whose probability of being a "bot" was located at the highest quartile of probability, which is > 0.975. When the algorithm was applied to the 469,616 users in the database, 69,033 accounts that could be defined as "bots" were detected. This amounts to a total of 15% of all accounts that were present in the digital debate. Similarly, the 69,033 bots posted 172,704 of the 1,208,631 messages in the filtered database, accounting for 14.28% of the total.
Polarisation measurement
It is important to remember that the calculation of polarisation uses the probabilities of being "for" or "against" the government that are offered by the machine learning algorithms as the degrees of membership of users to both poles, for or against. So, for the given individual "i," there are two values: 1) their probability of being in favour of the government and 2) their probability of being against the government. However, the "input" of the automatic classifiers are tweets, the objective being to calculate the polarisation of users. For each user, the average probability of being for and against the government was calculated for all their posted messages. Thus, for each user, the necessary two degrees of membership were obtained and could be used to calculate the polarisation measurement. On the other hand, due to the computational costs of applying the measure to 469,616 users, which meant comparing all users with each other, a total of (469,6162)/2=(469,6162)/2=110,269,593,728 JDJ calculations was necessary. The JDJ index was then calculated as the average of 1,500 JDJ iterations for a simple random sample of N=200 users per iteration.
First, polarisation was measured for the overall sample (“non-bots" and "bots"), obtaining a level of (JDJ_mean)1500=0.76; sd=0.027, resulting in a high level of polarisation JDJ_mean→[0,1]. Figure 1 shows the graphical representation of the distribution of users to be in favour of or against the government. A probability of 0.5 means to be against the government, while >0.5 means to be in favour.
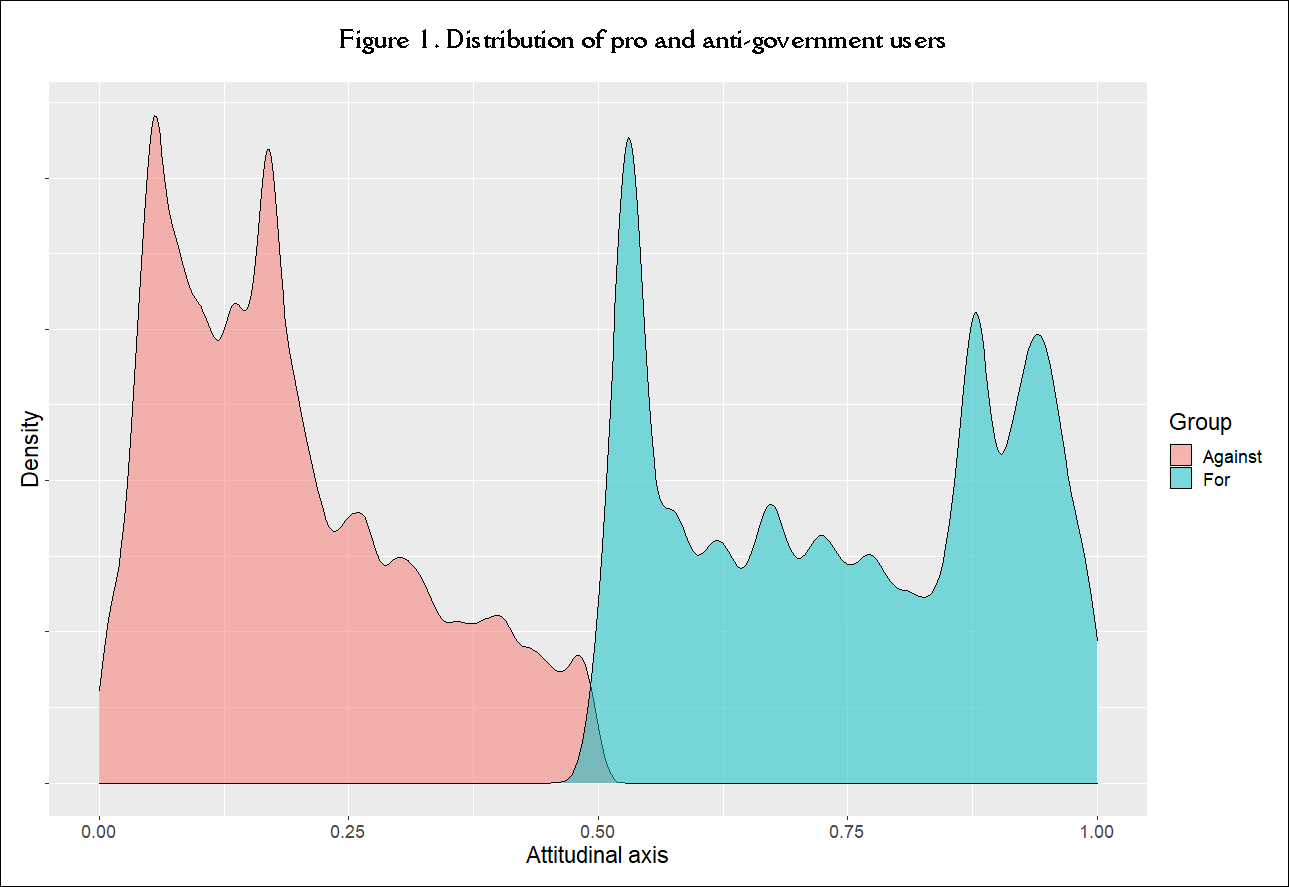
Polarisation levels were also calculated for 1) “non-bots” and 2) “bots”. Thus, JDJ showed an average polarisation level for the 1,500 iterations of JDJ_mean_no_bots1500=0.761; sd=0.026. For the "bots" group, it was JDJ_mean_bots1500=0.765; sd=0.026. Finally, the Mann-Witney test for two independent variables was applied to determine whether the differences found in the averages were statistically significant. Thus, with a statistic U=1021715, we found a significance level <0.000, finding a higher level of polarisation for "bot" users compared to “non-bot" users.
Detection of topics and polarisation
Firstly, the LDA topic detection algorithm was applied. Since the groups were established a priori, the algorithm was applied repeatedly by changing the parameter "number of groups" from one to ten to calculate the coherence for each number of clusters. It is important to remember that this coherence index is based on the semantic similarity between words. Therefore, after an expert had checked the algorithm’s different suggestions, the decision was made to opt for the detection of three topics (coherence of 0.42) in the dialogue since, as can be seen in Figure 2, they are well defined (health, economy, and politics). On the other hand, it is worth mentioning that due to the computational costs of the LDA algorithm, it was possible to access all the messages published by "bots" (N=172,704), while a simple random sample of N=200,000 was applied to the 1,035,927 messages from the group of “non-bots”. According to the formulas for calculating sample size (Fernández, 1996), with a population of N=1,035,927, a confidence level of 99% and a margin of error of 1%, the sample needed to be representative of the total was 16,378 messages. We therefore consider a simple random sample N=200,000 to be sufficient for achieving representativeness of the remaining messages from “non-bot” users.
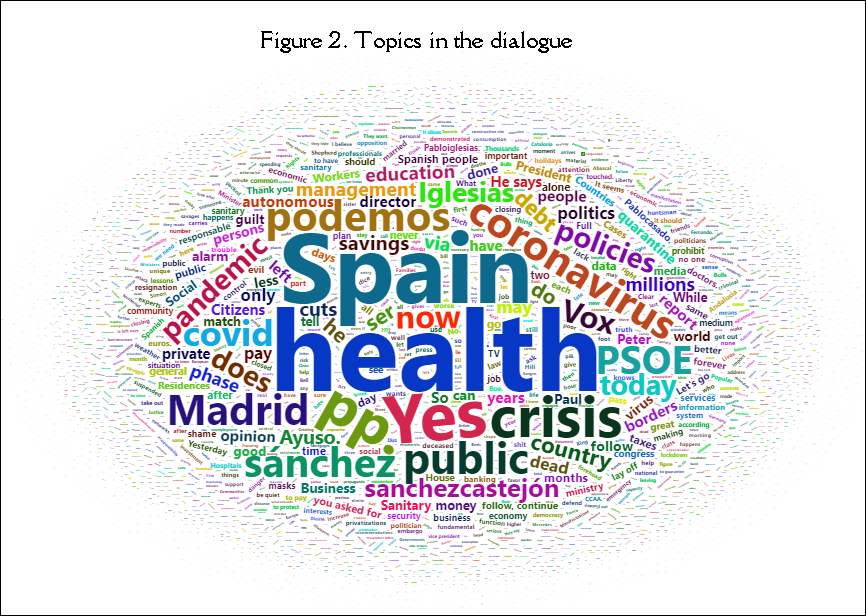
The number of topics found were the same for both groups ("bots" and “non-bots”), with “non-bot” users dominating the debate on economics (53.48%), followed by health (25.95%) and politics (20.55%), while for "bots" the main topic was politics (48.36%), followed by health (36.04%) and economics (15.58%).
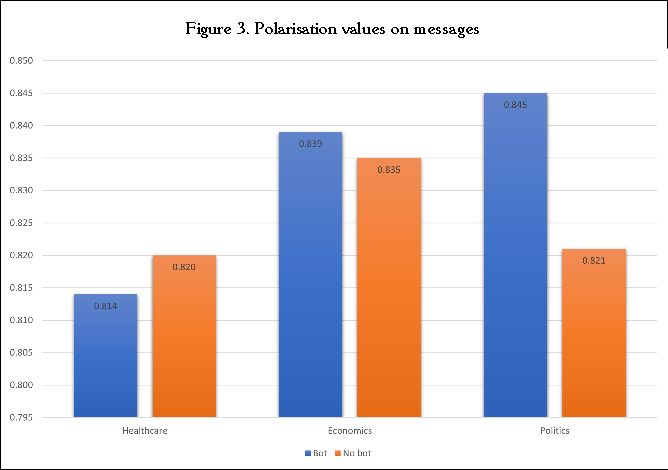
Once the topics were identified, the polarisation levels were calculated using JDJ. For this, the same procedure of calculating the average polarisation over 1,500 iterations for a random sample of N=200 per iteration was followed. Unlike the JDJ calculation in the previous section, here polarisation was calculated on messages and not on users. As can be seen in Figure 3, the highest polarisation values were found in messages produced by “bots”, more specifically those talking about politics (JDJ_mean1500=0.845) and economics (JDJ_mean1500=0.839), followed by messages posted by “non-bots” talking about economics (JDJ_mean1500=0.835). To find out whether the differences in levels of polarisation are statistically significant, a 2-factor ANOVA is performed: user ("bot" or “non-bot") x topic (health, economy and politics) (Table 2). Given the significance levels found (p<0.00), it can be concluded that both user type and subject matter affect polarisation levels. Furthermore, the interaction effect is also significant, which leads to the conclusion that the levels of polarisation found in the "topic" variable are conditioned by whether or not one is a "bot."
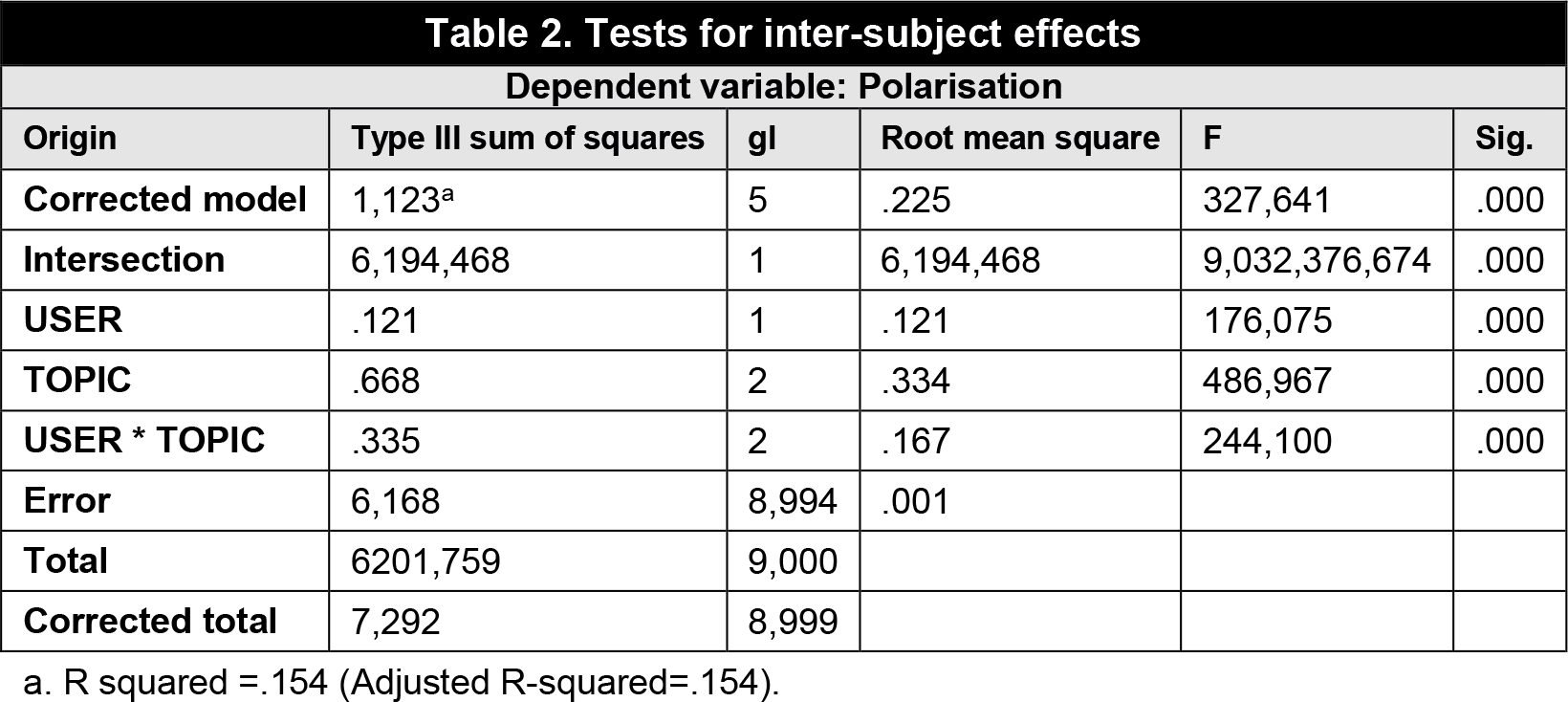
Finally, a new combination variable ("user" x "topic") with six levels was created to determine which of these scenarios had a higher level of polarisation. Thus, a one-factor ANOVA was applied, showing a statistic F(5)=327.641, p<0.000, where multiple comparisons were performed with Tukey's test, finding statistically significant differences between all levels except for the "no bot" - health and "no bot" - politics levels. It is safe to assume that the three highest levels of discourse polarisation were found in the political topic in the “bot” debate, followed by the economic topic in the “bot” debate and the economic topic in the “non-bot” debate (Figure 3).
Sentiment analysis and topics
Finally, the Afinn dictionary for sentiment analysis was applied to each of the detected topics. As can be seen in Figure 4, words with negative connotations predominate throughout the digital debate, finding a greater presence in messages that talk about the economy for the “non-bots” group (0.704%), followed by politics in the discourse of “bots” (0.687%) and politics in “non-bots” (0.672).
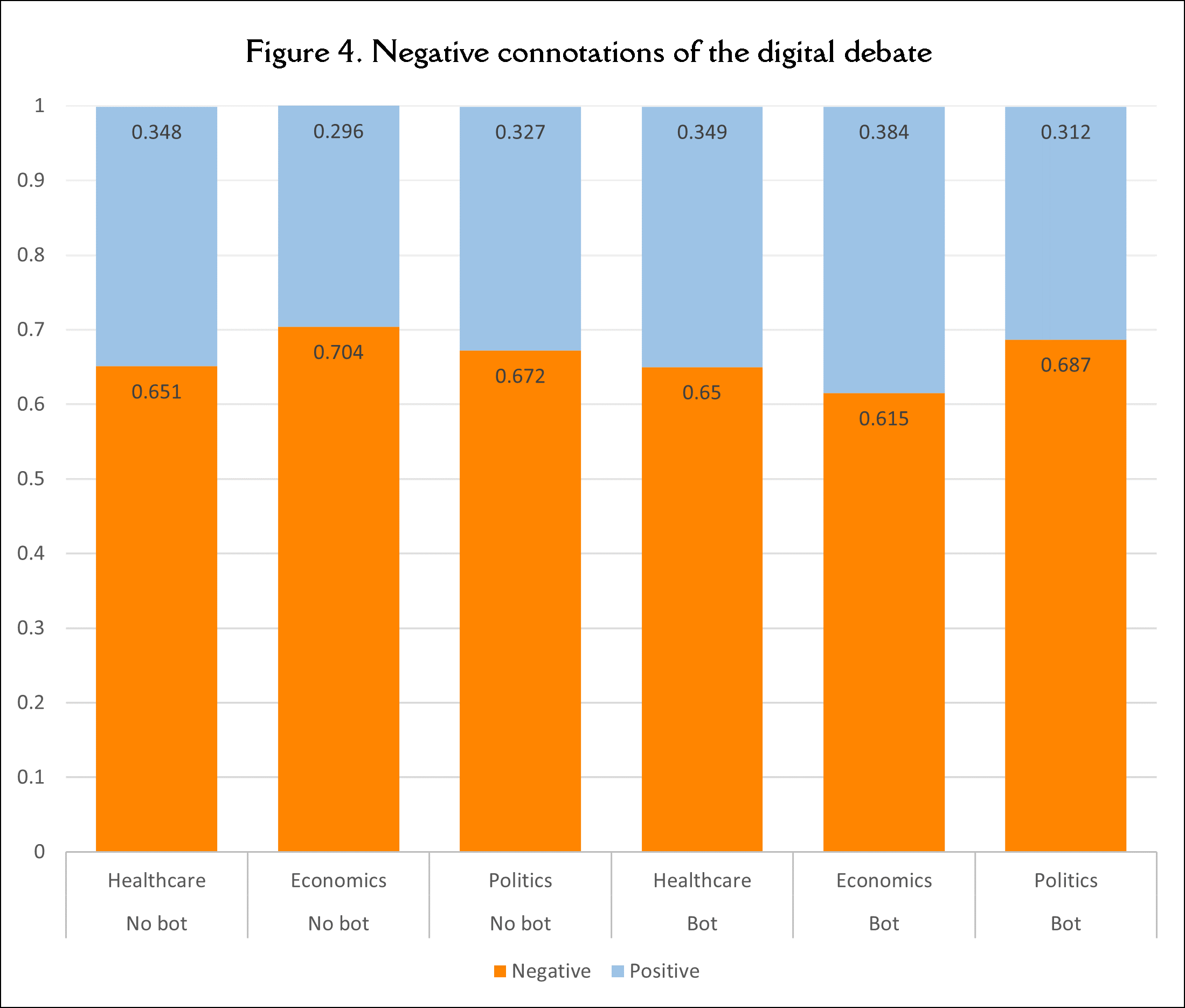
Discussion and conclusions
In this paper we set out to analyse to what extent and with what strategies political "bots" participate in the public discussion process through digital social networks. We focused particularly, as a sample case, on the Twitter debate surrounding the Spanish government's management of the global COVID-19 pandemic. The theoretical background of this research was to advance our understanding of a complex and potentially damaging communication process. That is, political polarisation and the emergence of a "communication failure" scenario. In short, we are talking about situations in which communication between participants tends to become fixated on strong viewpoints and to ignore, if not attack, those who think differently (“incivility”).
Many experts point to the presence of political polarisation in public debate processes, such as the one we are analysing here. However, the very definition of polarisation given in this article warns that this phenomenon does not depend solely on the existence of two or more opposing poles, but on a tendency towards isolation and a breakdown in communication between these different poles. A process derived from the so-called "echo chambers" on the web (Colleoni et al., 2014), in which information exchanges take place mainly between individuals with similar ideological preferences, especially when it comes to political issues (Barberá et al., 2015). Thus, we have shown (Figure 1) how the debate around governance was strongly polarised. In fact, our data suggests that the political “bots” identified in the analysis have a greater tendency to polarise public opinion than “non-bot” accounts. This finding is the necessary but not sufficient prelude to finding the type of polarisation that alerts us and encourages a rupture in communication.
The key factor (reason enough) for this rupture is, in our view, the identification of a strategy of distancing and entrenchment of the parties involved in the debate. Beyond the actions of political and media leaders and certain opinion leaders and thanks to sentiment analysis, in this study we have identified a strategy of negativisation of the debate that is more present among the "bots" than it is among the “non-bot” accounts. Our interpretation shows that this strategy seeks to skew opinion on the Spanish government's performance. It is biases of this kind that lead the way to polarisation in its most negative sense of rupture and distancing. An emotional polarisation that can have serious repercussions, particularly in times of political turmoil (Iyengar, 2019) such as that brought about by the onset of the pandemic.
This strategy becomes even clearer when we analyse the central topics of our case study. Here we observe how the "bots" tend to focus the debate on politics, rather than on economic or health issues (topics of debate that are potentially more subject to scientific and objectifiable criteria). This is a field in which it is easier to attack one or several figures rather than talk about general issues (as we have seen, the literature points to this strategy as a source of polarisation). In other words, it is an area in which it is more accessible to develop "ad hominem" strategies, represented in Figure 3 by the constant references to the Spanish Prime Minister, which are clearly more biased and focused on the flaws and the negative circumstances surrounding the person. In other words, it is an individual strategy centred on "incivility" as a form of communication management.
Political issues are also the most polarised in this debate. They also show the greatest difference between "bots" and “non-bots”. The latter present a more polarised debate on the management of the pandemic in political terms, being, out of the three topics, the one most negativised by the "bots". In line with the concept of outrage outlined by Sobieraj and Berry (2011), the topics found in the “bots” reinforce the melodrama and improbable predictions of impending doom that are attributed to decisions made by the government, a discourse distinguished by the tactics used to provoke emotion, rather than to evoke emotion in the political arena. Therefore, the use of "bots" does not seem to be oriented towards informing society about the risks of the pandemic or promoting prevention dynamics (Al-Rawi & Shukla, 2020), but rather is mostly focused on mobilising public opinion against the government by negativising it. As noted by Howard (2006), Walker (2014) and Keller et al. (2019), “bots” have the potential to influence users' political positioning online because, according to our results, they emulate ordinary citizens concerned with purely health issues. They can be considered political rather than social "bots" because they spread messages with negative sentiments (Stella el al., 2018; Neyazi, 2019; Yan et al., 2020; Adlung et al., 2021), specifically towards the government. These robots could have been designed to launch a political propaganda campaign of "astroturfing" initiated by traditional agents with the aim of increasing tension in a context of social emergency. Iyengar et al. (2019) warned that this is a type of strategy closely linked to the theory of the spiral of silence because, in a context of uncertainty and general frustration, it makes it difficult for users to express favourable opinions regarding any of the health measures taken by the government.
Our impression is that the polarisation-negativisation binomial is the ammunition chosen by these types of accounts to alienate and confront the parties involved in this public debate, as well as to create an environment of tension, lack of civility and attacks on those who think differently. In an already polarised context, whether it is due to the actions of other agents (political, social or media) or due to the situation of exceptionality and uncertainty itself, the ammunition used by the "bots" in this debate has consisted of making positions more extreme. This finding is one that can serve as a basis for future research and can be contrasted in various case studies with similar or different characteristics to the one carried out here.