(Un)founded fear towards the algorithm: YouTube recommendations and polarisation
Abstract
Social media have established a new way of communicating and understanding social relationships. At the same time, there are downsides, especially, their use of algorithms that have been built and developed under their umbrella and their potential to alter public opinion. This paper tries to analyse the YouTube recommendation system from the perspectives of reverse engineering and semantic mining. The first result is that, contrary to expectations, the issues do not tend to be extreme from the point of view of polarisation in all cases. Next, and through the study of the selected themes, the results do not offer a clear answer to the proposed hypotheses, since, as has been shown in similar works, the factors that shape the recommendation system are very diverse. In fact, results show that polarising content does not behave in the same way for all the topics analysed, which may indicate the existence of moderators –or corporate actions– that alter the relationship between the variables. Another contribution is the confirmation that we are dealing with non-linear, but potentially systematic, processes. Nevertheless, the present work opens the door to further academic research on the topic to clarify the unknowns about the role of these algorithms in our societies.
Keywords
Machine learning, YouTube, social media, recommendation system, polarisation, communication
Palabras clave
Aprendizaje de máquina, YouTube, redes sociales, sistemas de recomendación, polarización, comunicación
Resumen
Las redes sociales han instaurado una nueva forma de comunicarse y entender las relaciones sociales. A su vez, en lo que podría entenderse como un aspecto negativo, los algoritmos se han construido y desarrollado bajo el paraguas de un amplio abanico de conjeturas y diferentes posiciones al respecto de su capacidad para dirigir y orquestar la opinión pública. El presente trabajo aborda, desde los procesos de ingeniería inversa y de minado semántico, el análisis del sistema de recomendación de YouTube. De este modo, y, en primer lugar, reseñar un resultado clave, las temáticas analizadas de partida no tienden a extremarse. Seguidamente, y mediante el estudio de los temas seleccionados, los resultados no ofrecen una clara resolución de las hipótesis propuestas, ya que, como se ha mostrado en trabajos parecidos, los factores que dan forma al sistema de recomendación son variados y de muy diversa índole. De hecho, los resultados muestran cómo el contenido polarizante no es igual para todos los temas analizados, lo que puede indicar la existencia de moderadores –o acciones por parte de la compañía– que alteran la relación entre las variables. Con todo ello, trabajos como el presente abren la puerta a posteriores incursiones académicas en las que trazar sistematizaciones no lineales y con las que, tal vez, poder arrojar un sustento más neto y sustancial que permita despejar por completo parte de las dudas sobre el papel de los algoritmos y su papel en fenómenos sociales recientes.
Keywords
Machine learning, YouTube, social media, recommendation system, polarisation, communication
Palabras clave
Aprendizaje de máquina, YouTube, redes sociales, sistemas de recomendación, polarización, comunicación
Introduction and theoretical framework
The rise of ICTs has produced new scenarios both for social interaction and for the consumption of information and entertainment in societies at the end of the 20th century, especially in the first decades of the 21st century. In addition to the well-known mass media (including the press, radio, and television), other methods of disseminating and accessing messages on a mass scale have been added, which has also generated new symbioses in which the roles of the communication chain have tended to become blurred and hybridised (Berrocal-Gonzalo et al., 2014). Finally, we talk about the benefits and contributions of the Internet to democratise information dissemination mechanisms (Arias-Maldonado, 2016; Nielsen & Fletcher, 2020). While the Internet and its satellites were growing (boosted by the development of computing and technological advances in processing), so were the multiple studies on its effects. In this respect, this (inter)connected web that different authors (Berners-Lee, 2000), and with different adjectives (see McLuhan (1959), Habermas (1981) or Castells (2001) as a summary of these expressions), has engendered first the Web 1.0 and, later, the 2.0 (O'Reilly & Battelle, 2009), as well as other concepts (Latorre, 2022).
These developments give rise to various reflections on the effects they may have on citizens. Some of these new reflections already pointed to how the rise of the growing Web 2.0 could become the axis of a paradigm shift, ultimately posing a challenge and opportunity for both political spheres and liberal democracies in the 21st century (Sunstein, 2007; Lilleker & Jackson, 2008; Chadwick, 2009; Howard, 2021; Messina, 2022). However, the significant advances of the interconnected world were not yet fully consolidated. The emergence of what has come to be known as social media has led to a reconfiguration of the development of human relationships (Wigand et al., 2010). Thus, incorporating Facebook, Twitter, Instagram, or YouTube into everyday life has meant a change for contemporary societies, with users from all corners of the planet, as well as having a reach and diffusion ratio of more than a third of the world's population. Now that we are immersed in this transition period, the focus is on the effects that may arise from this new social and media drift driven by the networks. In this respect, part of the research carried out in academia and other spheres, such as journalism or politics, has focused on the internal side of interconnection. In this case, we are talking about the role that algorithms, especially their architectures and protocols, can play as mediators of the communication process.
Now, those related questions arise with the perspective of expanding algorithms. In this context, approaches emerge that point to the possible relationship between the role of social networks - although with special emphasis on their computational models - and the search for an understanding of singular social events: from a greater presence of political polarisation in public debate (Hernández et al., 2021), to situations that are complex to define, such as Brexit or the victory of Donald Trump in 2016. Despite the clear correlations that may exist between one event and another, the truth is that the research points to different sides without reaching conclusions. At least this is established in the doubts presented by works such as those of Rasmussen and Petersen (2022), Bail (2021) or Barberá (2020), who point to multifactorial, and even those who point to the analogical plane (Arceneaux & Johnson, 2010) as a key axis to reach an answer. This situation is therefore complex and subject to ambivalent dynamics. Based on this, the present research delves into some of the phenomena behind YouTube and its capacity to flood the multiple spheres of the media scene (Banaji, 2013). As Yesilada and Lewandowsky (2022) also point out, one of the critical factors focuses on the complexity of understanding its system. In this way, and as already pointed out by other studies, such as those of Luengo et al. (2021) or Serrano-Contreras et al. (2020), this paper aims to point out the drifts that the algorithm can generate. In addition, it seeks to incorporate into the debate whether this computational model is of any use in considering the emergence of social phenomena such as polarisation (Van-Bavel et al., 2021).
Data and method
This research proposes an analysis of the YouTube algorithm from multiple perspectives. In this regard, under the so-called reverse engineering process (Rekoff, 1985), as well as using text mining techniques and semantic measurement indexes, we seek to shape a progressive understanding of what lies beneath the computational architectures implemented by YouTube.
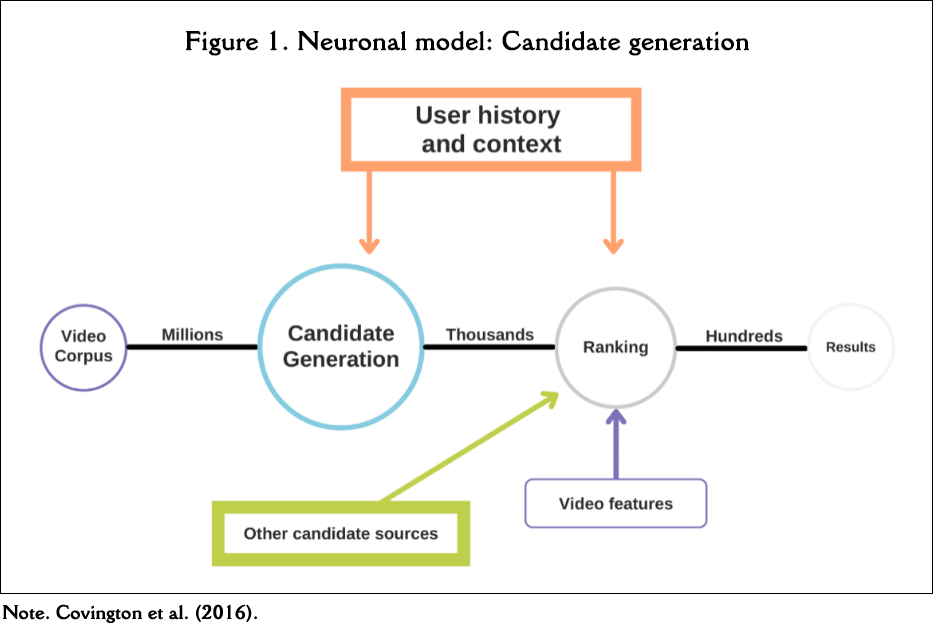
To this end, we seek to leave aside some of the main functions that Alphabet, as the owner of YouTube, incorporates into the training and subsequent development of the algorithm of its video server (see the work of Alphabet's employees, Davidson et al. (2010) or Covington et al. (2016), as well as Figure 1, for an approximation of the model that the platform uses to offer the results to the user). In this way, the goal is to try to parameterise the behaviour of the model avoiding the set of passive and active data that we provide on the network while browsing (e.g. location; search history; personal data...), since all these metrics are used to compile detailed information about our supposed interests - fundamental to our usage experience (Dimopoulos et al., 2013); On the other hand, we seek to review the model. These experiences are the basis for assumptions which are indispensable for understanding some of the most commonly used nomenclatures when discussing algorithms, such as the bubble filter (Pariser, 2017) and other externalities (Bishop, 2018).
Within this set of effects that the algorithmic era can causethere is a factor that has become very popular recently, the idea of radicalisation. To support these ideas about the reinforcement of a position, we used work, research, and empirical examples, which have shown how the platform's algorithm tended to become more and more extreme (Tufekci, 2018; Alfano et al., 2021; Almagro & Villanueva, 2021; Chen et al., 2021).
Therefore, the behaviour of YouTube's recommendation algorithm, based on users' interests, should lead to higher consumption of related materials. In other words, it will interpret users' searches as interests and thus try to make it easier for them when searching, by recommending similar videos (which would result in a filter bubble). It follows that recommendations could result in greater polarisation by causing less exposure to different viewpoints or topics. Hence, the first hypothesis we propose in this research is:
-
H1: Videos recommended by YouTube will become increasingly extreme or polarised.
At the same time, such recommendations could create communities with very similar interests (called homophilic classifications), which, in turn, could be related to echo chambers. Therefore, our second research hypothesis is:
-
H2: Comments on videos recommended by YouTube will be equally polarised.
The logic of the research is that, according to part of the scientific community 1 , YouTube's algorithm recommendations move towards extrapolating the interests raised by users' searches, creating this filter bubble and, at the same time, increasing the polarisation of the content shown (by being increasingly focused on very specific content or a specific point of view). Users should thus behave similarly.
Figure 2 describes the research we have designed to test the hypotheses. First, we selected an existing YouTube account belonging to one of the researchers, which had never been used (we could say that there was no initial metadata linked to the account, so the searches we initiated would create the metadata about our interests) 2 . Subsequently, we chose topics on which to apply the design. We tried to ensure relevant topics that served to discriminate specific moderators that could affect the relationship between the variables we intended to analyse.
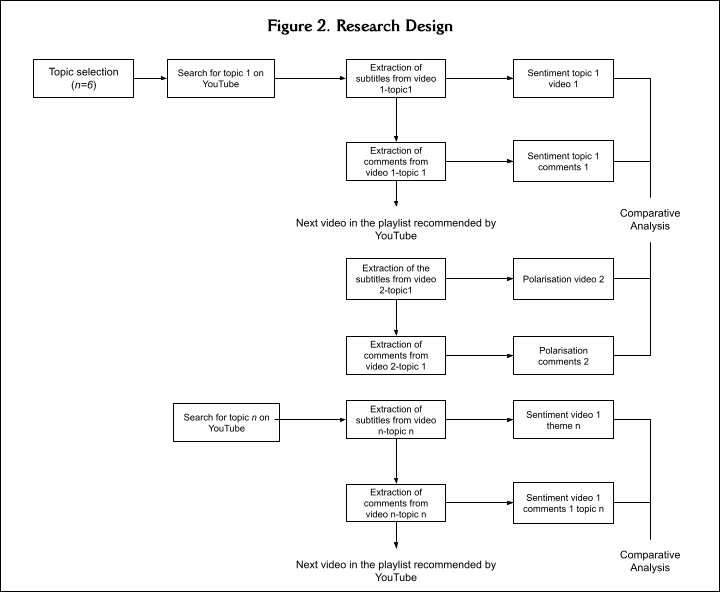
Thus, the topics 3 were:
-
(Spanish) National politics: search for "política nacional."
-
(Spanish) Political parties at ideological extremes: searches for "Podemos" and "Vox."
-
Vegetarianism: search for "comida vegetariana."
-
Conflict: search for "guerra en Ucrania."
-
Feminism: search for "feminismo."
-
COVID: search for "COVID."
In other words, topics differed in conflict and were related to various fields, from conflict to vegetarianism. All were sensitive topics where we expected significant comments (on the videos that allowed it).
The next step was to start searching for the topic on YouTube, both on the proposed account and without any account, and analyse the first video that the results recommended. When the video was played, we continued with the automatic playback. Thus, we could see the recommendation made by YouTube's algorithm and up to a certain number of videos (with a maximum of 100 per topic). So, we have at least two first videos per topic, one searching with the account and the other without. The goal was to see whether the algorithm behaved differently in the absence of account metadata.
The videos were analysed by extracting their content through the subtitles (therefore, it is an analysis of the textual script, not the images). As this is a resource that is not present in all the videos on the platform, it was decided to apply the analysis to one video out of every 10 in the playlist (or the one that was closest in ordinal order), provided that there was a sufficient number of videos (as will be explained, in the case of the videos captured without an account on the platform, the text was extracted from all the videos that contained it). With this text, we proceeded to an affective polarisation analysis (on the debate around the concept, see Iyengar et al., 2019) using a technique that we had used on previous occasions with considerable success (see Serrano-Contreras et al., 2020, for a detailed explanation). The procedure consisted of modifying a sentiment analysis (the selected tool was Orange3, Demsar et al., 2013, based on Python and using a multilingual dictionary for more than 50 languages). The modification consisted of calculating the mean sentiment of a given dataset and measuring the distance between the unit of analysis and the overall sample (thus being an affective distance analysis). In this case, we varied the analysis to measure the polarisation of videos and comments based on the sentiment of the first video by topic and account. Thus, the polarisation of the other videos and comments is given as the distance from the first one, but in absolute numbers. This way, we can tell if a particular video has a tone that is distant (positive or negative) from the first one. We do not look at how negative or positive the videos are, as these are circumstantial considerations and would require a detailed analysis of the content, but only at their distance from the first one. We understand that affective polarisation can occur through positive and negative charges (for example, cheering or congratulating a terrorist group). The same analysis was done concerning comments, except all possible comments were analysed, including videos without subtitles (although not all videos allow comments). The numerical results range from 0 to 100, but it is common to obtain very low numbers (around 0.1-2). This is because most of the content is not affectively loaded (even after pre-processing the text with the usual techniques, as was done). The only consequence is that minor changes in value indicate substantial variations in affective polarisation; after all, we are talking about millions of analysed words (Table 2).
For each video, then, the following variables were extracted: those provided by the platform (number of likes, views, number of comments, etc.), topic, position in the automatic playlist (platform recommendation), polarisation of the video’s content (one out of ten in those extracted with an account) and polarisation of the video’s comments (in those that have them enabled). Finally, tables 1 and 2 describe the number of units of analysis (750 videos and, including comments, nearly three million words).
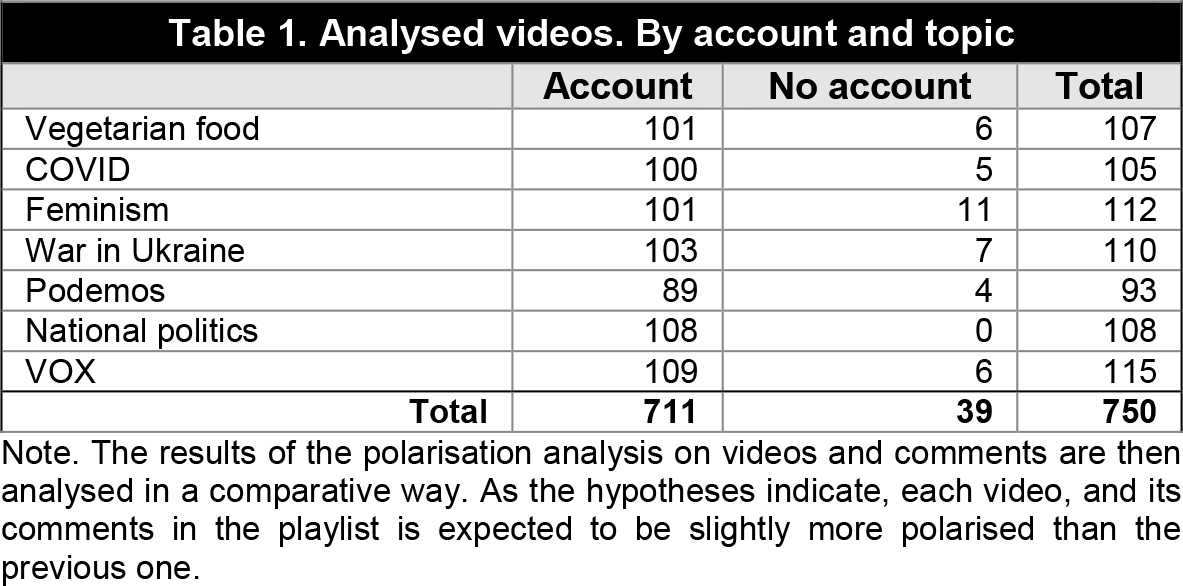
Findings
One element that needs to be highlighted is that YouTube changes its parameters and ways over time. Therefore, in this research, we could not work with both "likes" and "dislikes," as only positive data is now provided. However, we do not believe that this will affect the research.
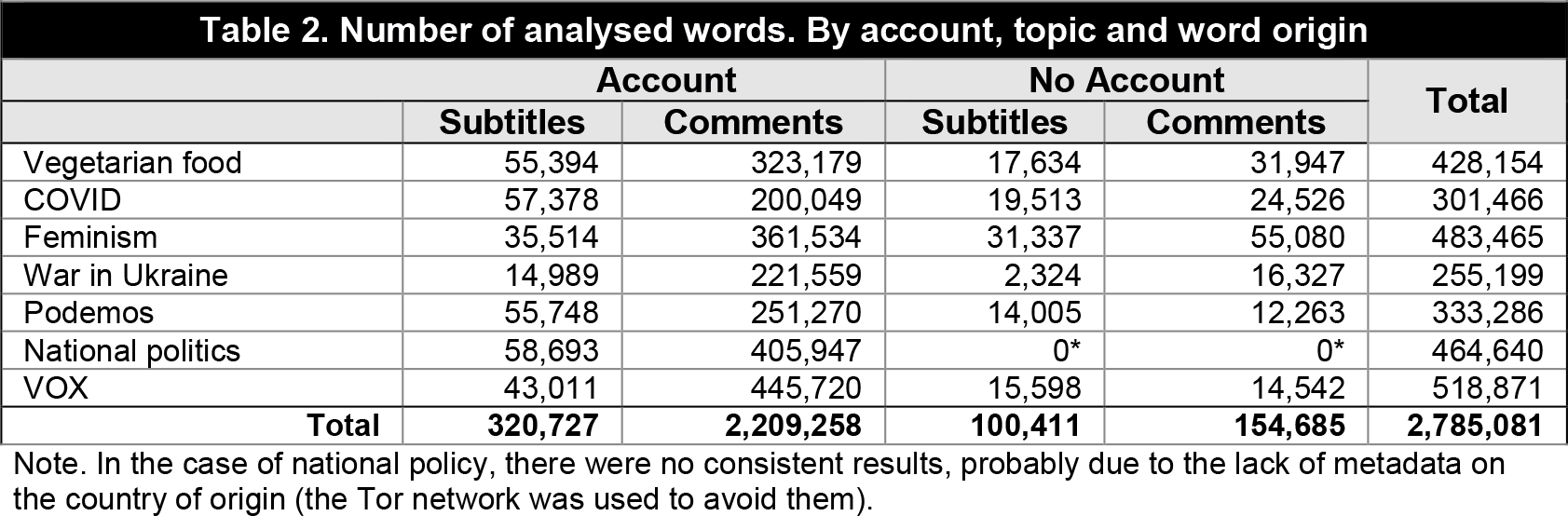
Along the same lines, YouTube does not provide the exact same content to one user as to another, something that is evident from what has already been outlined by Pariser (2017) and from daily consumption. However, there is another fact to take into consideration. Logging in with or without an account produces different results (Table 1). Despite the obvious, there is another interesting element that has been found in this work: when an automatic playback is carried out, if the process is conducted with an account, the model continues to offer videos, but if it is done without an account, the model ends up entering a loop in which two videos tend to playrepeatedly. Hence, the need to place a limit on the 100th video is unnecessary in the case of access without an account. This also affects the length of the videos. While the length of the videos did not seem to be a factor without an account and have set a constant consumption, the model tended to offer longer and longer videos.
Another fact to consider, and already noted in the evolution from Davidson et al. (2010) to Covington et al. (2016), is that YouTube's ranking of results varies over time. This seems to be evidenced by the type of content offered by autoplay. This is seen in both the topic of feminism and vegetarianism. While the former seems to be influenced by the fact that a video from the TED talks channel was selected in the first instance, in the latter, even though it could generate opposing positions such as vegetarianism, the type of video, in this case, based simply on cooking recipes, has meant that the algorithm has not tended towards other paths as has happened with the rest of the topics, which bifurcated and diverted to other areas. For example, in the case of the conflict in Ukraine, a large part of the final sample is made up of relaxing music videos. Thus, there are factors that the algorithm aims to reward in order to filter a certain content to offer the user. This position seems to be a clear commitment by the company (see also Goodrow (2021) and Mohan (2022) for a detailed explanation of the actions undertaken by the platform to create content that is less harmful for both information and consumption by users of all age ranges). Hence, Table 2 shows data that can sometimes be paradoxical, such as the fact that there are more words analysed from subtitles than from comments.
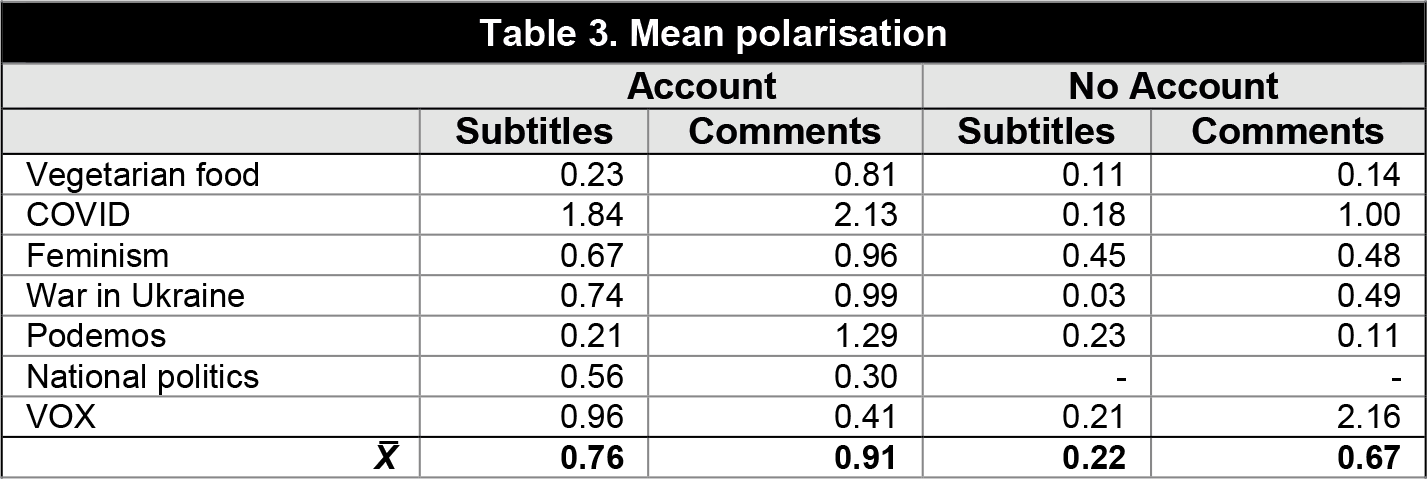
Let us look at the average aggregate polarisation data (Table 3). It is easy to observe the different values depending on the topics, the accounts, and whether they come from the videos themselves or the comments. In the first case, there clearly are topics where polarisation is higher, especially those referring to "COVID" (X̅=1.35), followed by "VOX" (0.68), “national politics" (0.56) and "feminism" (0.55). Although results are not surprising, there are essential differences between those analysed with and without an account (especially in the case of "VOX" and "COVID").
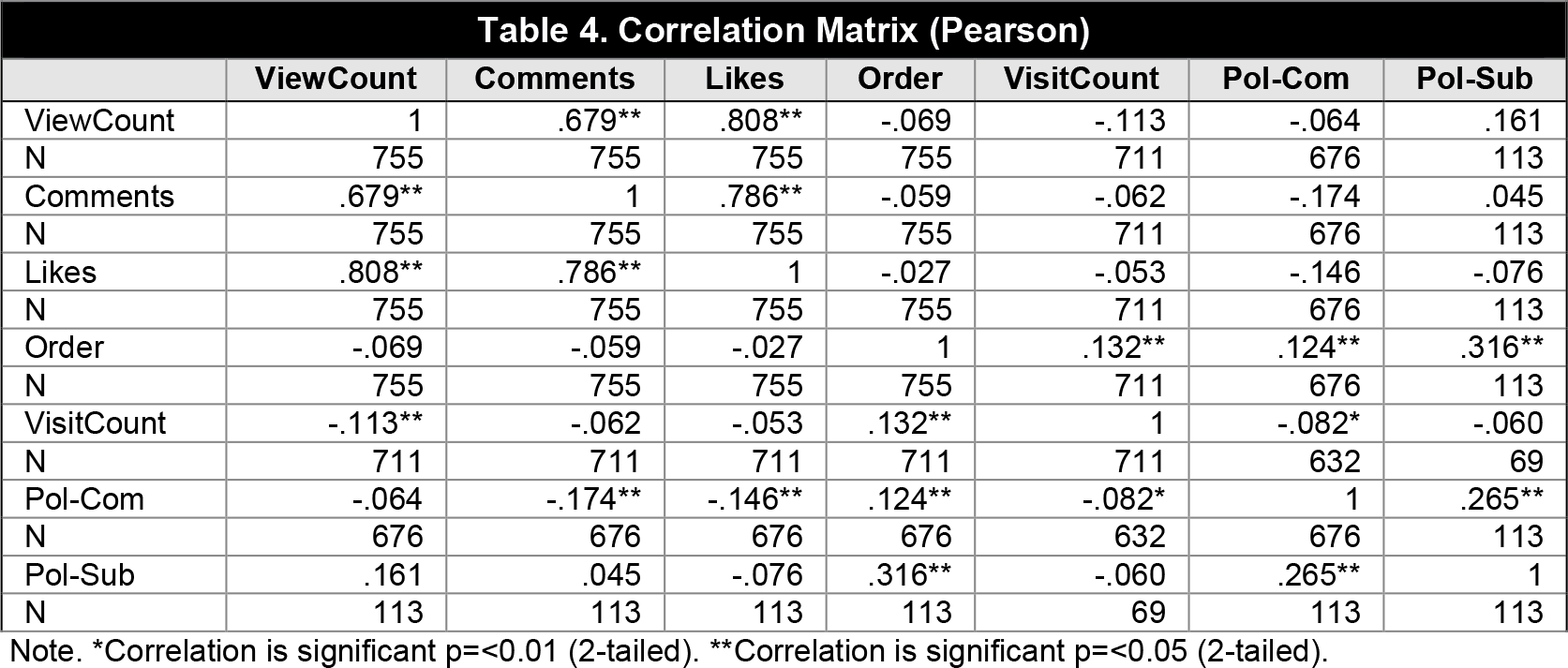
Except for "COVID" (2.07), user comments do not show a similar pattern, with "war in Ukraine" (0.95), "Podemos" (0.95) and "feminism" (0.88), in addition to the aforementioned "COVID," being the topics where the most significant polarisation has been observed. Although there are also notable differences concerning the origin of the video (with or without an account), the data seem to point to the existence of a possible echo chamber in some cases or, at least, to a certain degree of agreement between users with an account who comment on the videos we have catalogued as "VOX" and "national politics," while those who comment on the videos captured with the searches "COVID," "Podemos" or "Ukrainian war," show signs of highly polarised comments. Overall, the videos analysed with a Google account have an average polarisation of 0.76 with a fairly high dispersion (+- 0.70), while those analysed without it have a much lower polarisation of 0.22 (+-0.25). The distance is smaller if we include the polarisation in the users' comments, 0.91 and 0.67 respectively; in both cases, it is substantially higher than that obtained from the videos.
As can be seen in Table 4, the correlation matrix provides exciting data. On the one hand, we have the expected correlations, such as the relationship between likes, comments, and views; the correlations are robust because, in essence, they are measuring the same thing: the popularity of a given video. On the other hand, the variable we are most interested in is "Order," which indicates the position in the playlist offered by YouTube, and this is where there are interesting findings. Fundamentally, we can see a positive correlation between polarisation and the order of the video, both in the comments (Pol-Com) and in the video itself (Pol-Sub) and between themselves. Indeed, this is not an excessively strong relationship, but it is not negligible either, especially the relationship between the polarisation of the video and the order (.316).
With regards to video polarisation, it is interesting to explore this more closely. The relationship between the two variables is not linear (the results of attempting to model it using linear regression have been unsuccessful, x2=-0.002), so it is likely there is a moderator. It was decided to analyse the means to discover the variable that may be altering this relationship and to recode the Order variable into three segments (Order-Cat): one to three videos, four to nine, and more than nine. The segmentation is, of course, not arbitrary. We have estimated that it is possible and even likely, that a user will watch up to three videos proposed by YouTube in a row. We find it less likely that between four and nine videos will be watched and quite unlikely that more than nine videos in a row suggested by the algorithm will be watched. Thus, we consider that, in the first case, we would face a low exposure to the algorithm, medium in the second, and high in the third.
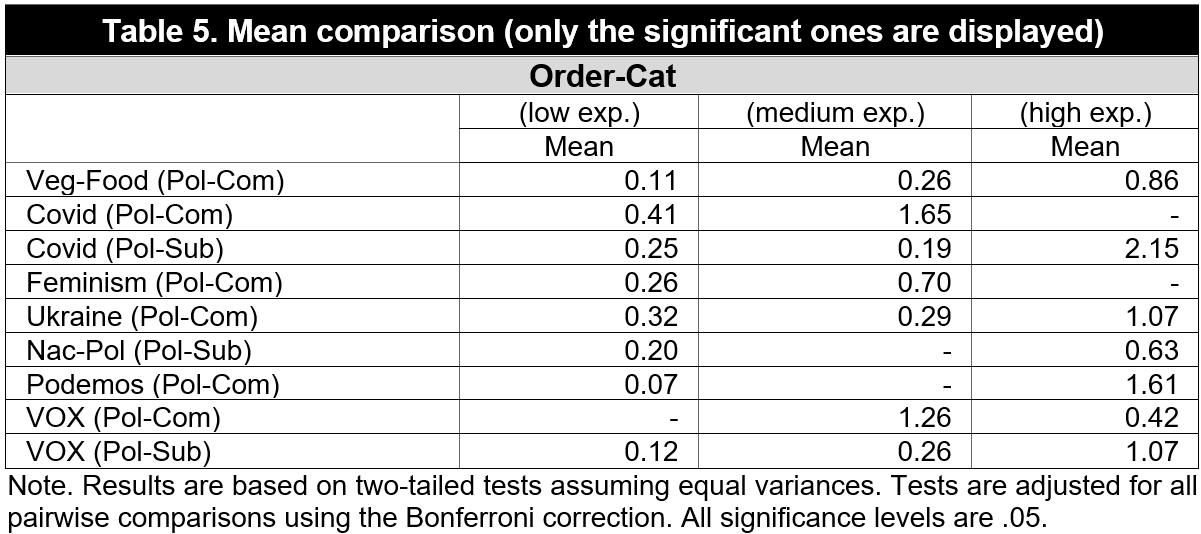
Table 5 shows the mean comparison results, although only in those cases where the test indicates significant differences. The first impression is that each topic seems to behave differently: the videos (Pol-Sub) offered by the platform when searching for the terms "COVID", "national politics", and "VOX", indeed tend to be more polarised in each of the three proposed sections (except the first to the second of "COVID"). The case of "VOX" is perhaps the clearest and it shows how the average polarisation increases in each section in an almost linear fashion. In regard to comments, the topics where differences can be seen are "vegetarian food," "COVID," "feminism," "Ukrainian war," "Podemos," and "VOX." In other words, on all topics. However, unlike the case of the videos, we observe different trends: while in all of them, the tendency continues to be towards an increase in polarisation, the opposite is observed in VOX.
In the case of both the videos and the comments, we expected a similar relationship between the different topics. However, the differences are so substantial that it is challenging to validate the initial hypotheses. There does appear to be some relationship between the position of the video - and its comments - and an increase in polarisation, but the exceptions prevent us from claiming a direct relationship. Perhaps some particularly prominent cases are being modified by the company itself, as with COVID, where an effort was made to ensure that citizens received less extreme information. In any case, results indicate moderators that are difficult to measure in this relationship.
Discussion and conclusions
Approaches to the debate on the role of algorithms are slowly taking hold. Despite this, although the various positions are beginning to make clear outlines of what we may face, other aspects are still challenging to address. This is the case with recommendation systems (Yesilada & Lewandowsky, 2022). In this particular area, works such as the present one, hopefully, serve to portray what happens in processes such as those of YouTube. At least through this sampling model, it seems evident that the chosen themes do not tend to become extreme. In other words, playing a video about vegetarian food does not end up with a video about veganism or "anti-speciesist" movements. That circumstance seems to be exposed to more complex factors than the simple issue of search, not least the ability of YouTube's training model to access user data and interests and generate a consumption pattern. This, in turn, it is a variable to be considered when carrying out data collection actions with this series of sampling methods. However, we cannot give a conclusive answer. As shown at the beginning, theorists state that recommendation algorithms may be causing the phenomena called filter bubbles and echo chambers (Terren & Borge-Bravo, 2021). However, the results presented here are not conclusive: there are topics where the algorithm does seem to behave in that direction, but there are other topics where such a relationship, at least with our research strategy, is not perceived.
Nevertheless, the results offered are striking and consistent with previous research, where we found that the topic strongly moderates content polarisation and user reactions (Serrano-Contreras et al., 2020). This opens up other questions worthy of further research: to find whether topics are a moderator in this relationship. For these topics, could we claim that these hypotheses hold? And, more importantly, why? Unfortunately, the data offered here are insufficient to answer these questions beyond the description of those chosen. However, they humbly contribute to the direction that the relationship, if it exists, is neither direct nor linear. Nevertheless, the results may be different with a selection based on other groupings, e.g., topics on politics, extremism, music, etc., as well as implementing coherent monitoring of clusters of so-called prosumers. Moreover, with the proper internal coherence, conclusions that move towards the definitive answer to the question that theorists have been asking for a decade or more could be reached: do social networks polarise our citizens? (1)